The landscape of AI has witnessed a revolutionary shift with the evolution of Large Language Models (LLMs). These sophisticated algorithms, capable of understanding and generating human-like text, have transformed how we interact with technology. At the forefront of this evolution is Zephyr-7B, a model that stands out not just for its size but for its remarkable efficiency and performance.
Developed by the innovative team at Hugging Face, Zephyr-7B is a testament to the rapid advancements in AI. Born from the foundations laid by its predecessor, Mistral 7B, Zephyr-7B exemplifies the pinnacle of what smaller, more efficient models can achieve. It's not just another model in the sea of AI; it's a beacon of efficiency and effectiveness, setting new standards in the realm of LLMs.
The Birth of Zephyr 7B Models
Zephyr 7B Alpha: The Pioneer
Zephyr 7B Alpha marked the initial foray into the world of fine-tuned language models. Here's what sets it apart:
- Foundation Model: Zephyr 7B Alpha is a fine-tuned version of Mistral 7B, renowned for its status as one of the best small open-source pretrained models.
- Training Method: The training process for Zephyr 7B Alpha involved three key stages:
- Distilled Supervised Fine-Tuning (dSFT): This phase began with the construction of a large-scale, self-instruct-style dataset called UltraChat, followed by distilled SFT.
- AI Feedback (AIF) Collection: Four different large language models (LLMs) generated completions, which were then ranked by GPT-4 to collect feedback (UltraFeedback).
- Distilled Direct Preference Optimization (dDPO): The dSFT model was refined using feedback data through DPO.
- Overfitting Insights: Interestingly, overfitting on the preference dataset during DPO yielded better chat results, highlighting the model's adaptability.
Zephyr 7B Beta: Refined and Enhanced
Zephyr 7B Beta builds upon the foundation laid by its alpha predecessor, with several notable improvements:
- Benchmark Performance: Zephyr 7B Beta's performance in benchmark tests is a standout feature. It scored 7.34 on MT-Bench, surpassing Llama 2 Chat 70B's score of 6.86. However, it achieved a slightly lower win rate of 90.60% on AlpacaEval, compared to Llama 2 Chat 70B's 92.66%.
- Direct Preference Optimization (DPO): Zephyr 7B Beta continues to utilize DPO, but it refines and enhances this approach, resulting in improved chat results.
- Training Focus: Beta version training places a strong emphasis on achieving alignment with human preferences, a key factor in enhancing the model's performance.
Before you dive into the world of Zephyr 7B, you might want to get a taste of what it can do. The team has provided an interactive demo where you can engage with the model and witness its conversational prowess. Give it a try Anakin AI!
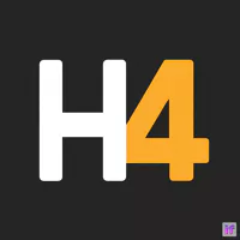
What Sets Zephyr 7B Beta Apart?
Just as with its alpha release, Zephyr 7B Beta distinguishes itself not only through metrics but also through its unique training process and insights gained along the way.
- Fine-Tuning with Purpose: Zephyr 7B Beta continues the tradition of fine-tuning a strong foundation. It starts with the Mistral 7B model, ensuring that the core capabilities are robust and reliable. This approach allows Zephyr to build upon a solid foundation, enhancing its conversational abilities.
- Data for Training: UltraFeedback, the large-scale preference dataset, remains a cornerstone of Zephyr 7B Beta's training. By understanding and prioritizing user preferences, Zephyr 7B Beta ensures that its responses align with what users expect, creating a more satisfying conversational experience.
- Shift to Direct Preference Optimization (DPO): ย Zephyr 7B Beta retains its commitment to Direct Preference Optimization (DPO), a method that streamlines the fine-tuning process. By using feedback data directly, DPO eliminates the need for a reward model, making the training process more efficient and effective.
- Overfitting for Better Chat Results: A fascinating insight that carries over from Zephyr 7B Alpha is the concept of overfitting. Contrary to conventional wisdom, overfitting on the preference dataset has proven to yield better chat results. Zephyr 7B Beta embraces this phenomenon, demonstrating its adaptability and capacity to learn from user interactions.
- Using Supervised Fine-Tuning (SFT): As demonstrated in the alpha phase, Zephyr 7B Beta reaffirms that both Supervised Fine-Tuning (SFT) and Direct Preference Optimization (DPO) are essential for success. The synergy between these techniques ensures that the model not only understands but excels in the art of conversation.
Zephyr 7B Beta represents a significant leap forward, building upon the successes of its alpha counterpart. It's not just a model; it's a conversational partner that continues to learn and adapt, striving for excellence in every interaction.`
Benchmarks for Zephyr-7B Beta
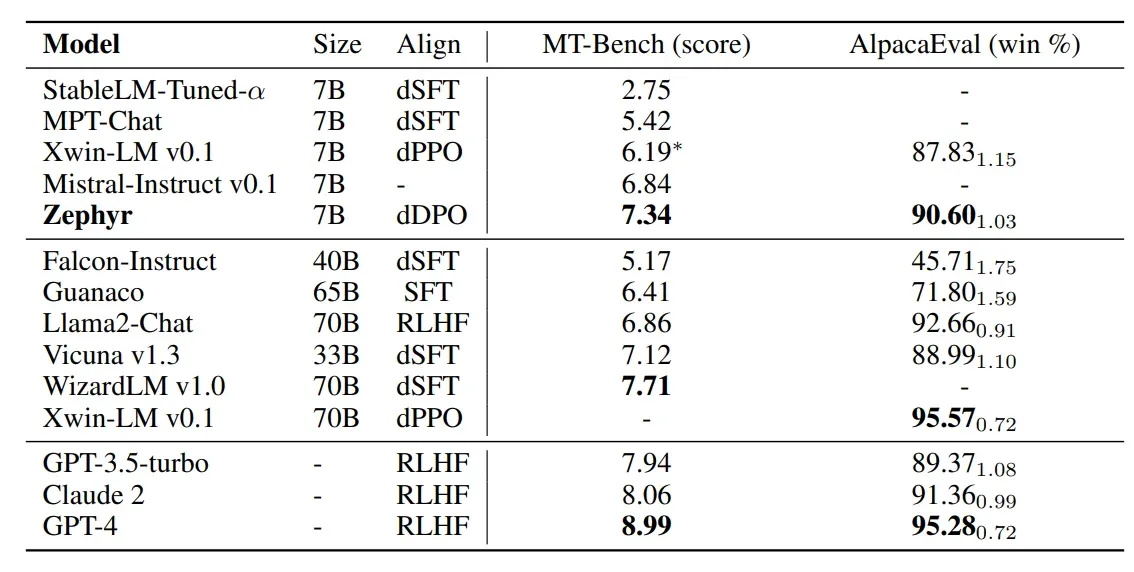
Zephyr 7B Alpha and Beta have made significant strides in benchmark performance. The beta version, in particular, stands out with its ability to excel in complex conversation scenarios. Here is the radar chart for model comparison:
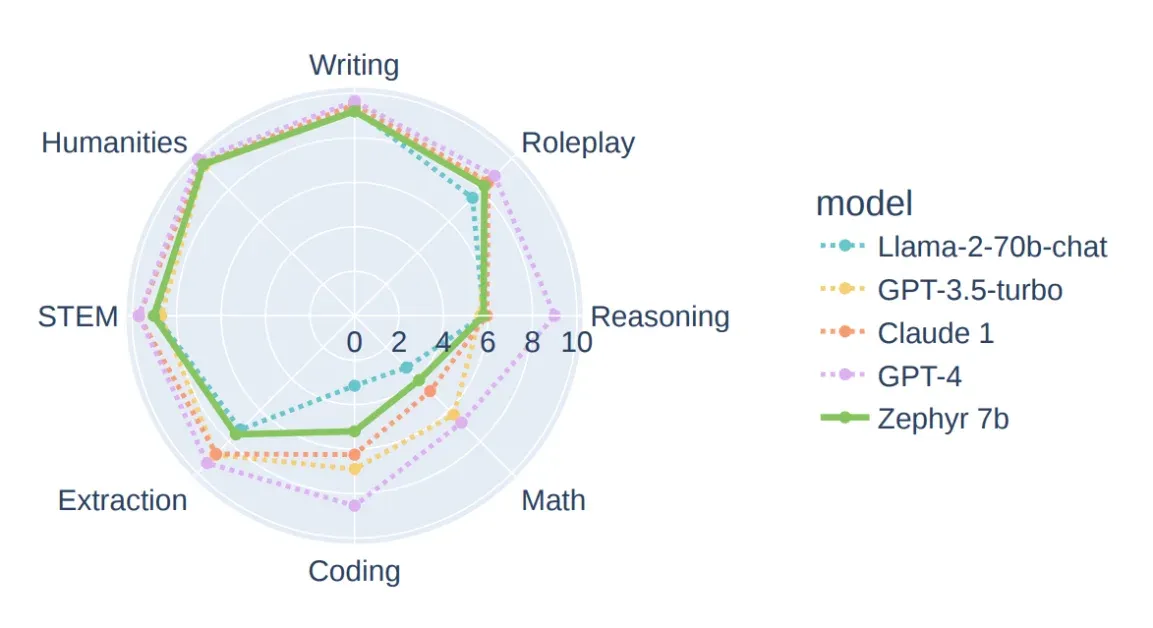
To place Zephyr 7B in context, let's compare its performance with other notable models:
- GPT-4: In comparison to GPT-4, Zephyr 7B Beta exhibits impressive benchmark scores. While GPT-4 may have a slightly higher score in some metrics, Zephyr Beta's focus on alignment and efficiency make it a compelling competitor.
- Llama 2 Chat 70B: Zephyr 7B Beta outshines Llama 2 Chat 70B in the MT-Bench, highlighting its prowess in handling multi-turn conversations. Although Llama 2 Chat maintains a slight edge in AlpacaEval, Zephyr Beta's overall performance is commendable.
Fascinated about Zephyr 7B's performance? Test it out on Anakin AI!
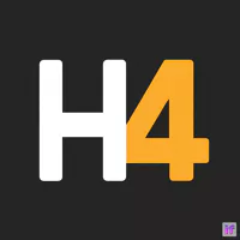
The Training Stages of Zephyr 7B Models, Explained:
- Distilled Supervised Fine-Tuning (dSFT): Zephyr's journey to excellence begins with a foundation in the form of the Distilled Supervised Fine-Tuning (dSFT) stage. During this phase, the model immerses itself in a vast and diverse collection of dialogues known as the UltraChat dataset. It learns in a self-instruct style, absorbing the nuances of language and conversation.
- AI Feedback (AIF) Collection: The second stage introduces the concept of collaborative learning. Four distinct, large language models contribute by generating potential responses. These responses are then subjected to evaluation by the formidable GPT-4. This process results in the creation of the UltraFeedback dataset, which serves as a valuable resource for further refinement.
- Distilled Direct Preference Optimization (dDPO): The final stage, Distilled Direct Preference Optimization (dDPO), represents the pinnacle of Zephyr's training. Here, the model undergoes the transformative power of DPO, using the wealth of feedback data acquired in the previous stage. This refinement process leads to significant enhancements in the quality of conversations that Zephyr can engage in.
The Outcome of Training Approaches
- Overfitting Works! One of the remarkable discoveries in Zephyr's journey is the unexpected advantage of overfitting on the preference dataset. Counterintuitively, this overfitting has proven to be a catalyst for improved conversational capabilities across various benchmarks. Zephyr's ability to tailor its responses based on preferences has set it apart.
- SFT and DPO, Combined: Experiments and rigorous testing have underscored the pivotal role played by both Supervised Fine-Tuning (SFT) and Direct Preference Optimization (DPO). It has become evident that the combination of these two training approaches is the secret sauce that makes Zephyr shine in chat scenarios. DPO alone may not suffice; it is the synergy of SFT and DPO that elevates Zephyr's performance.
Zephyr Beta vs Alpha, What are the Improvements?
Zephyr Alpha, while impressive, wasn't without its quirks.
- Users highlighted issues with casing, where responses occasionally featured incorrect letter capitalization.
- Some responses had peculiar prefacing, affecting the overall flow of the conversation.
Zephyr Beta takes these insights to heart and has undergone additional filtering to address these concerns. As a result, users can expect more natural and seamless interactions with Zephyr Beta, marking a step forward in its evolution.
How to Run Zephyr 7B with Hugging Face Transformers
You can easily run Zephyr 7B using the Hugging Face Transformers library. Here's a guide on how to run the model with sample code:
# Import necessary libraries
import torch
from transformers import pipeline
# Create a pipeline for text generation with Zephyr 7B Beta
pipe = pipeline("text-generation", model="HuggingFaceH4/zephyr-7b-beta", torch_dtype=torch.bfloat16, device_map="auto")
# Define the chat messages
messages = [
{
"role": "system",
"content": "You are a friendly chatbot who always responds in the style of a pirate",
},
{"role": "user", "content": "How many helicopters can a human eat in one sitting?"},
]
# Apply the chat template and generate a response
prompt = pipe.tokenizer.apply_chat_template(messages, tokenize=False, add_generation_prompt=True)
outputs = pipe(prompt, max_new_tokens=256, do_sample=True, temperature=0.7, top_k=50, top_p=0.95)
# Print the generated response
print(outputs[0]["generated_text"])
This code sets up a chat with Zephyr 7B Beta, where you can define the role of the chat participants and input your questions or messages. The model will generate responses in accordance with the defined roles and content. Feel free to customize the messages and parameters as needed for your specific use case.
Limitations of Zephyr 7B
While Zephyr 7B Beta is a powerful language model, it comes with certain limitations and considerations:
Safety Concerns: Zephyr-7B-ฮฒ has not undergone alignment to human preferences for safety within the RLHF phase. This means that the model can produce problematic outputs, especially when prompted to do so.
Filtering of Responses: Unlike models like ChatGPT, Zephyr-7B-ฮฒ is not deployed with in-the-loop filtering of responses. Users should exercise caution when using the model to ensure appropriate and safe interactions.
Training Data: The exact size and composition of the corpus used to train the base model (Mistral-7B-v0.1) are unknown. It is likely that the training data included a mix of web data and technical sources such as books and code. This can impact the model's knowledge and responses.
It's essential to be mindful of these limitations when using Zephyr 7B Beta and consider the context in which it is applied.
Conclusion
In summary, Zephyr 7B Alpha and Zephyr 7B Beta are cutting-edge language models that have surpassed benchmarks, thanks to innovative training techniques like DPO. These models represent a significant leap in conversational AI and offer exciting possibilities for natural language processing tasks. While they excel in various scenarios, users should be mindful of potential limitations and responsible usage to ensure safe and ethical interactions.
You can easily test out the power of Zephyr 7B Models on Anakin AI:
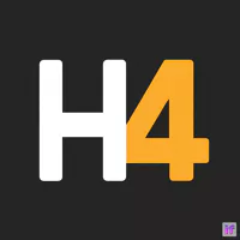
Want to test out more awesome LLMs online? Try Anakin AI!
Anakin AI is one of the most convenient way that you can test out some of the most popular AI Models without downloading them!
Here are the other Open Source and Free Models that Anakin AI supports:
- Mistral 7B and 8x7B: the hottest names for Open Source LLMs!
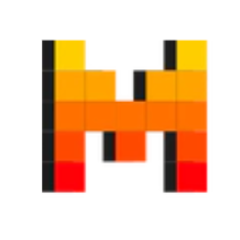
- Dolphin-2.5-Mixtral-8x7b: get a taste the wild west of uncensored Mixtral 8x7B!
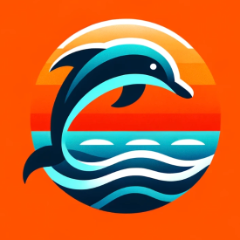
- OpenHermes-2.5-Mistral-7B: One of the best performing Mistral-7B fine tune models, give it a shot!
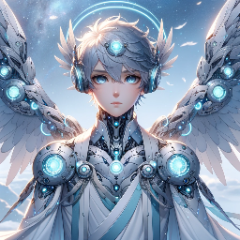
- OpenChat, now you can build Open Source Lanugage Models, even if your data is imperfect!
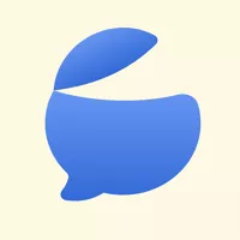
Other models include:
- GPT-4: Boasting an impressive context window of up to 128k, this model takes deep learning to new heights.
- Google Gemini Pro: Google's AI model designed for precision and depth in information retrieval.
- DALLE 3: Create stunning, high-resolution images from textual descriptions.
- Stable Diffusion: Generate images with a unique artistic flair, perfect for creative projects.
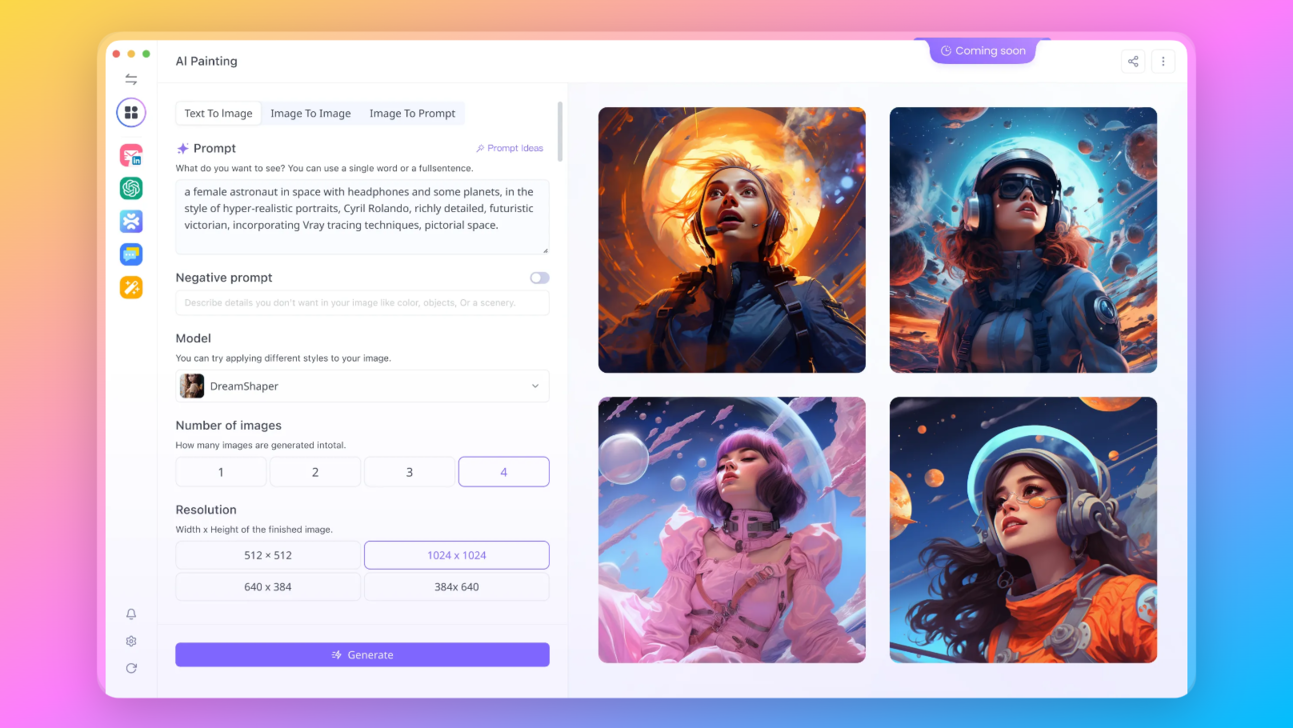
Want to test out all these awesome LLMs online? Try Anakin AI!
Anakin AI is one of the most convenient way that you can test out some of the most popular AI Models without downloading them!