Meta's Llama 3.1 series represents a significant leap forward in the realm of large language models (LLMs), offering three distinct variants: the massive 405B parameter model, the mid-range 70B model, and the more compact 8B model. This article provides a comprehensive comparison of these three models, focusing on their performance benchmarks and pricing considerations.
Anakin AI is your go-to solution!
Anakin AI is the all-in-one platform where you can access: Llama Models from Meta, Claude 3.5 Sonnet, GPT-4, Google Gemini Flash, Uncensored LLM, DALLE 3, Stable Diffusion, in one place, with API Support for easy integration!
Get Started and Try it Now!๐๐๐
Llama 3.1 405B vs 70B vs 8B: Models Overview
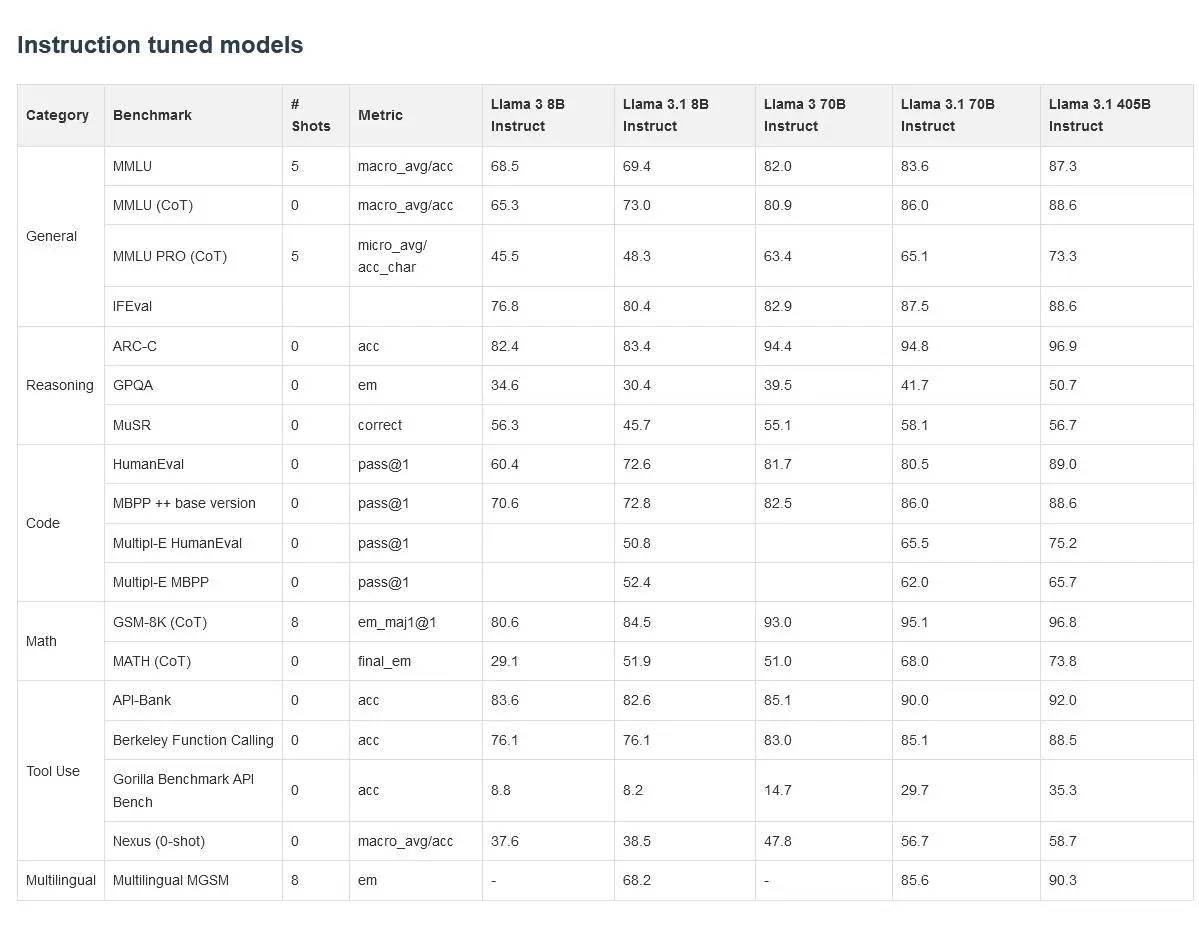
The Llama 3.1 series builds upon the success of its predecessors, introducing improvements in multilingual capabilities, reasoning, and overall performance. All three models share some common characteristics:
- Training Data: Trained on 15T+ tokens from publicly available sources
- Multilingual Support: Explicit support for French, German, Hindi, Italian, Portuguese, Spanish, and Thai
- Architecture: Based on an optimized transformer architecture
- Context Length: 128k tokens for all models
Let's dive into the specifics of each model variant.
Performance Benchmarks of Llama 3.1 405B vs 70B vs 8B
To understand the capabilities of each model, we'll examine their performance across various benchmarks. The following table provides a comparison of key metrics:
Benchmark | Llama 3.1 8B | Llama 3.1 70B | Llama 3.1 405B |
---|---|---|---|
MMLU | 66.7 | 79.3 | 85.2 |
MMLU PRO (CoT) | 37.1 | 53.8 | 61.6 |
AGIEval English | 47.8 | 64.6 | 71.6 |
CommonSenseQA | 75.0 | 84.1 | 85.8 |
Winogrande | 60.5 | 83.3 | 86.7 |
BIG-Bench Hard (CoT) | 64.2 | 81.6 | 85.9 |
ARC-Challenge | 79.7 | 92.9 | 96.1 |
TriviaQA-Wiki | 77.6 | 89.8 | 91.8 |
SQuAD | 77.0 | 81.8 | 89.3 |
DROP (F1) | 59.5 | 79.6 | 84.8 |
Key Observations:
- Consistent Improvement: Across all benchmarks, we see a clear pattern of improvement as we move from the 8B to the 70B and finally to the 405B model.
- Significant Jumps: The leap from 8B to 70B often shows a more substantial improvement compared to the jump from 70B to 405B, suggesting diminishing returns at higher parameter counts.
- 405B Dominance: The 405B model consistently outperforms its smaller counterparts, often by a significant margin, especially in complex reasoning tasks like MMLU PRO and AGIEval.
Instruction-Tuned Performance
The instruction-tuned versions of these models show even more impressive results:
Benchmark | Llama 3.1 8B Instruct | Llama 3.1 70B Instruct | Llama 3.1 405B Instruct |
---|---|---|---|
MMLU | 69.4 | 83.6 | 87.3 |
MMLU (CoT) | 73.0 | 86.0 | 88.6 |
MMLU PRO (CoT) | 48.3 | 65.1 | 73.3 |
ARC-C | 83.4 | 94.8 | 96.9 |
HumanEval | 72.6 | 80.5 | 89.0 |
GSM-8K (CoT) | 84.5 | 95.1 | 96.8 |
MATH (CoT) | 51.9 | 68.0 | 73.8 |
Key Insights:
- Instruction Tuning Boost: All models show significant improvements when instruction-tuned, with the gap between base and instruct versions being particularly noticeable for the 8B model.
- 405B Excels in Complex Tasks: The 405B model shows its strength in tasks requiring deep reasoning and mathematical abilities, such as MATH and GSM-8K.
- Diminishing Returns: While the 405B model consistently outperforms the others, the gap between 70B and 405B is smaller than that between 8B and 70B in many benchmarks.
Pricing Considerations
Pricing is a crucial factor when considering which model to use for various applications. Here's a breakdown of the known pricing information:
- Llama 3.1 405B: Estimated monthly cost between $200-250 for hosting and inference
- Llama 3.1 70B: Approximately $0.90 per 1M tokens (blended 3:1 ratio of input to output tokens)
- Llama 3.1 8B: Specific pricing not available, but expected to be significantly lower than the 70B model
Cost-Effectiveness Analysis:
- 405B Model: While offering the highest performance, its high computational requirements make it suitable primarily for enterprises and large-scale applications. The cost may be justified for tasks requiring top-tier performance.
- 70B Model: Represents a good balance between performance and cost. It's significantly more powerful than the 8B model while being more accessible than the 405B variant.
- 8B Model: Likely the most cost-effective option for many applications, especially where budget constraints are a primary concern.
Deployment Considerations
The size and computational requirements of these models play a crucial role in their deployment:
405B Model:
- Requires specialized hardware (e.g., multiple DGX systems with 8xH100s)
- May be offered in both FP16 and FP8 versions, with FP8 potentially being more cost-effective
- Not suitable for consumer-grade GPUs
70B Model:
- Can be deployed on high-end GPU systems
- More accessible for medium to large organizations
8B Model:
- Most versatile in terms of deployment options
- Can potentially run on consumer-grade GPUs with appropriate optimizations
What's the Best Use Case for Llama 8B, 70B and 405B?
Based on the performance benchmarks and pricing considerations, here are some recommendations for different use cases:
Enterprise-Level Applications:
- Best Choice: 405B model
- Reasoning: Offers top-tier performance for complex tasks, suitable for organizations with substantial computational resources
Mid-Size Companies or Research Institutions:
- Best Choice: 70B model
- Reasoning: Balances high performance with more manageable computational requirements
Startups or Individual Developers:
- Best Choice: 8B model
- Reasoning: Offers good performance for many tasks while being the most accessible in terms of cost and hardware requirements
Multilingual Applications:
- Recommendation: Consider the larger models (70B or 405B) for better performance across multiple languages
Resource-Constrained Environments:
- Best Choice: 8B model, potentially with quantization
- Reasoning: Can be optimized to run on less powerful hardware while still providing decent performance
Conclusion
The Llama 3.1 series offers a range of models to suit different needs and resources. The 405B model stands out as a powerhouse, rivaling and sometimes surpassing proprietary models like GPT-4o in certain benchmarks. However, its deployment challenges and cost make it suitable primarily for large-scale applications.
The 70B model strikes a balance between performance and accessibility, making it an attractive option for many organizations. It offers significant improvements over the 8B model while being more manageable than the 405B variant.
The 8B model, while less powerful, remains a viable option for many applications, especially where cost and deployment flexibility are primary concerns. Its performance is still impressive for its size, and it can be a great starting point for many projects.
Ultimately, the choice between these models will depend on the specific requirements of the task at hand, available computational resources, and budget constraints. As the field of AI continues to evolve rapidly, we can expect further improvements and optimizations that may shift the balance between these models in the future.
Anakin AI is your go-to solution!
Anakin AI is the all-in-one platform where you can access: Llama Models from Meta, Claude 3.5 Sonnet, GPT-4, Google Gemini Flash, Uncensored LLM, DALLE 3, Stable Diffusion, in one place, with API Support for easy intergration!
Get Started and Try it Now!๐๐๐