Text generation models like GPT-4 have revolutionized natural language processing, allowing us to generate human-like text effortlessly. However, these models can sometimes produce outputs that are either too repetitive or too nonsensical.
Tip: The "top-p" parameter is located to the right side of the OpenAI Playground.
This is where the "top-p" parameter comes into play. In this article, we will unravel the mystery of the top-p parameter and understand how it impacts text generation in the OpenAI Playground.
Key Points in this Article:
- Explaining the role of the top-p parameter in text generation.
- Understanding how the top-p parameter influences the diversity of generated text.
- Comparing the top-p parameter with the top-k parameter for controlling text diversity.
Let's dive in and demystify the top-p parameter.
Want to test out the ChatGPT powered AI Agent?
Use Anakin AI for creating any AI Agent at your will!
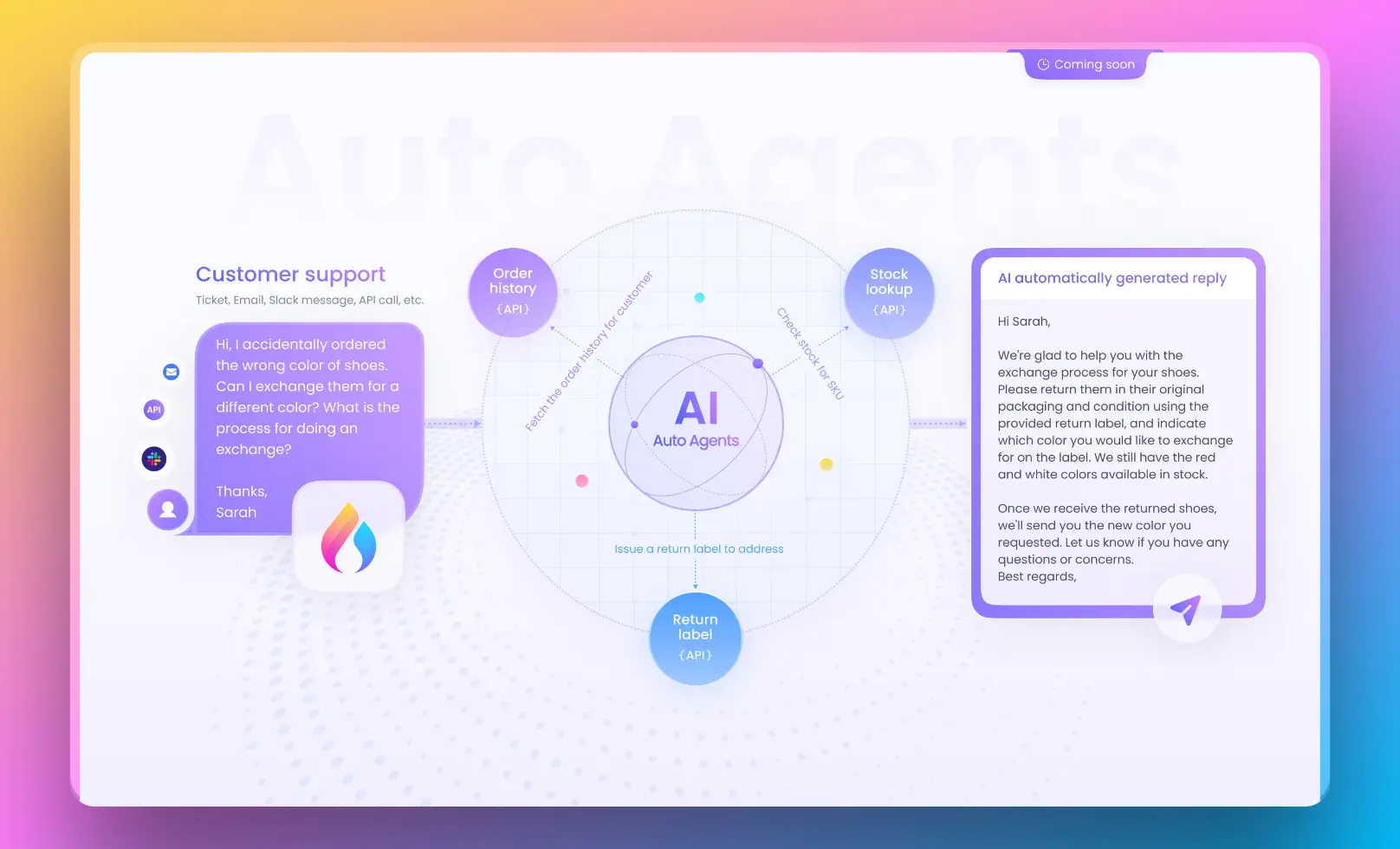
What is the Top-p Parameter in OpenAI Playground?
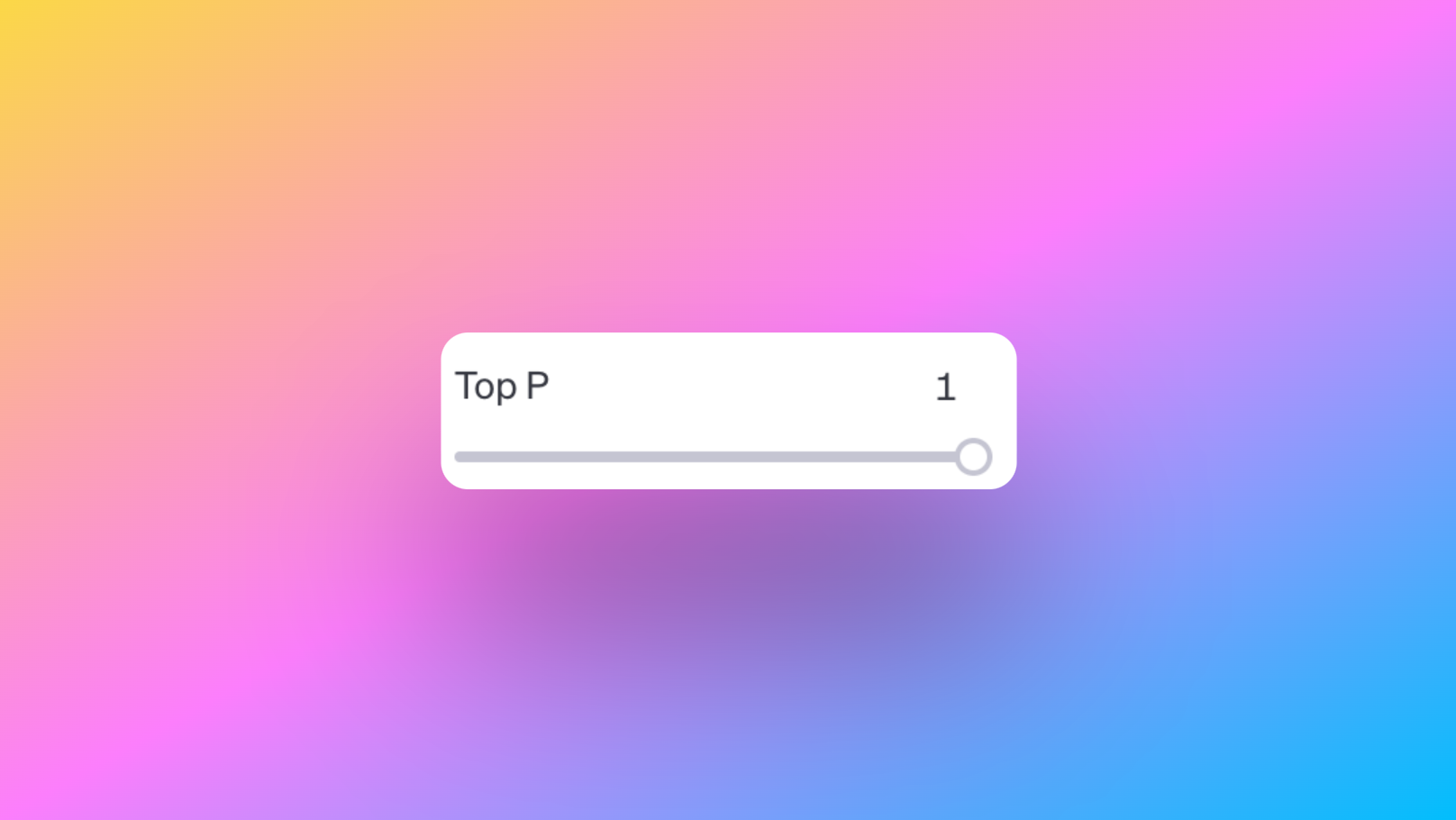
At its core, the top-p parameter is a critical component in text generation models, particularly in the context of GPT-4. Here's what you need to know:
- Function: The top-p parameter serves as a threshold for the cumulative probability of the most likely tokens that can be included in the generated text.
- Diversity Control: One of its primary functions is controlling the diversity of generated text. A lower top-p value leans towards selecting more common and repetitive words, resulting in less diverse output. Conversely, a higher top-p value allows the model to consider a broader range of tokens, leading to more diverse but potentially nonsensical output.
- Risk Modulation: Think of the top-p parameter as a risk modulator. It enables users to fine-tune the "risk" the model takes when generating text. Lower values favor more predictable and focused output, while higher values favor more diverse but potentially less coherent output.
- Balancing Act: The top-p parameter is all about balance. It helps users strike a harmonious equilibrium between text diversity and relevance to the given context. This ensures that the generated output aligns with the user's requirements and stylistic preferences.
- Recommendations: OpenAI typically recommends using either temperature sampling or nucleus sampling, but not both, in conjunction with the top-p parameter. The choice of the top-p value can be adjusted based on specific use cases and desired output quality.
In essence, the top-p parameter is a powerful tool for fine-tuning text generation. It empowers users to tailor the output to meet their specific needs, whether they require highly focused content or are in pursuit of creative diversity.
How the Top-p Parameter Shapes Text Diversity
Now that we've grasped the essence of the top-p parameter, let's delve deeper into how it influences the diversity of the text generated by AI models. The relationship between the top-p value and text diversity can be summarized as follows:
- Diversity Control: The top-p value plays a pivotal role in controlling the diversity of generated text. Higher top-p values result in more diverse and creative outputs, as they allow the model to consider a broader range of tokens, including less probable ones. Conversely, lower top-p values lead to more focused and deterministic text, as they restrict the model to consider only the most probable tokens[1][4].
- Risk Modulation: Consider the top-p parameter as your risk knob. When you increase the top-p value, you turn up the risk by encouraging the model to explore less probable tokens, which can lead to more diverse but potentially nonsensical output. Conversely, decreasing the top-p value reduces the risk, leading to more predictable but less diverse output[1][4].
- Balance Between Diversity and Relevance: It's all about finding the sweet spot. By adjusting the top-p value, users can finely balance the level of diversity and relevance in the generated text. This ensures that the output not only showcases creativity but also remains coherent and contextually appropriate.
In summary, the top-p parameter grants users the power to modulate text diversity based on their specific requirements. Whether you seek creative diversity or precise relevance, the top-p parameter is your ally in achieving the desired text output.
What is the Difference Between Top-p and Top-k Parameters
In the realm of text generation, you may encounter another parameter known as "top-k." While both top-p and top-k parameters contribute to controlling text diversity, they operate in distinct ways. Here's how they differ:
Top-p Parameter:
- Top-p sampling dynamically selects from the smallest possible set of words whose cumulative probability exceeds the probability p. This means that the size of the set of words can change based on the probability distribution.
- Higher top-p values result in more diverse output, as they allow the model to consider a wider range of tokens, including less probable ones. Lower values lead to more focused and deterministic text.
Top-k Parameter:
- In contrast, top-k sampling focuses on considering only the k most likely next tokens at each step of text generation. It directly limits the number of tokens under consideration.
- Lower top-k values concentrate the sampling on the highest probability tokens, resulting in less diverse output, while higher top-k values enable the model to consider a larger number of tokens, leading to more diversity.
In essence, both top-p and top-k parameters play vital roles in balancing text diversity and relevance. Users can choose between them based on their specific preferences and use cases, adjusting the values to achieve the desired level of control over text generation.
What About Other OpenAI Parameters?
Temperature: Shaping Creativity
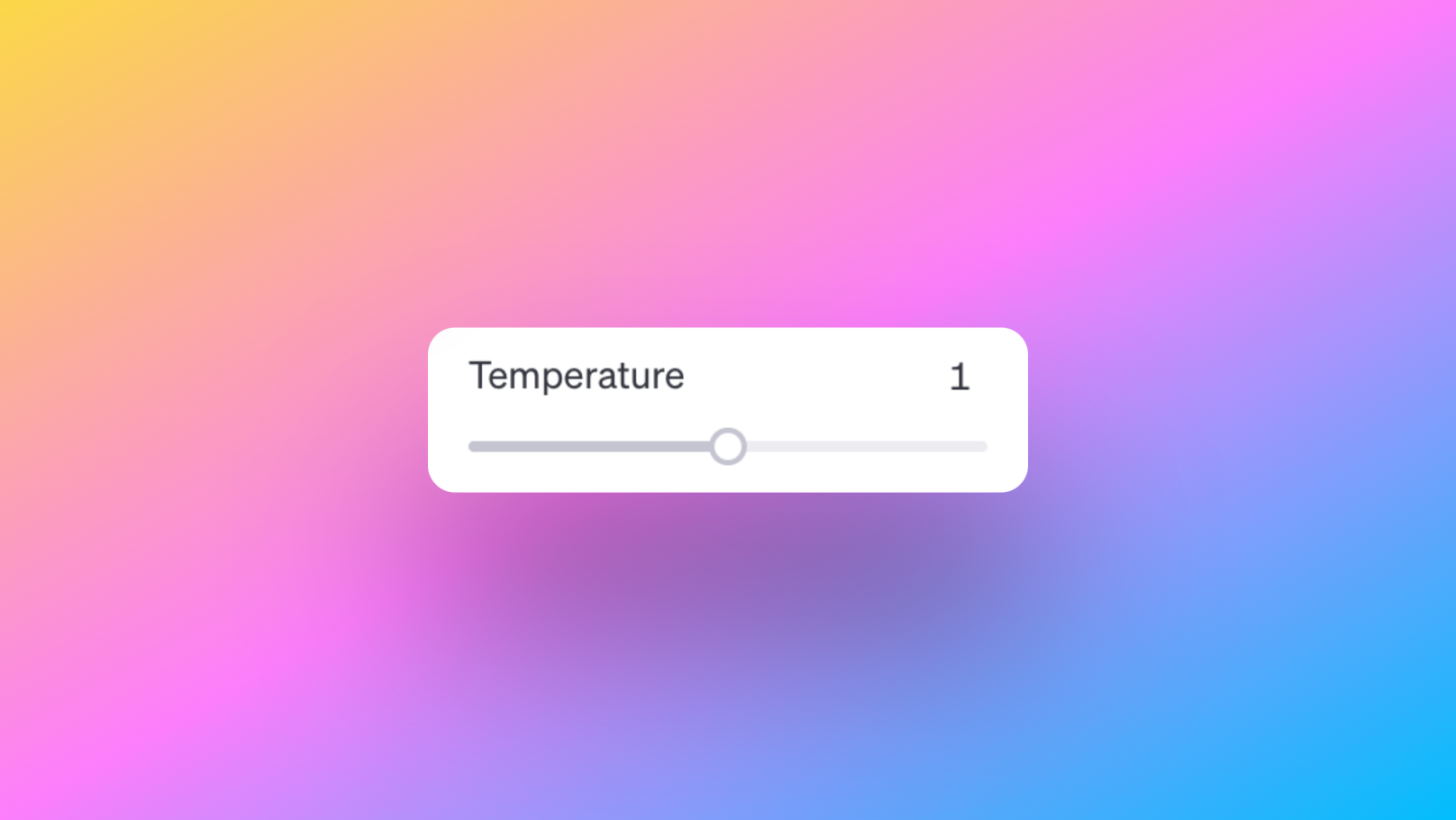
Temperature is a critical parameter when it comes to controlling the creativity and randomness of the generated text. It acts as a creativity knob, and here's how it works:
- High Temperature (e.g., 1.0): When you set the temperature to a higher value, the model becomes more creative and generates diverse output. This means it's more likely to select words that are less probable, leading to imaginative and unpredictable text.
- Low Temperature (e.g., 0.2): Conversely, a lower temperature value makes the model more focused and deterministic. It leans towards selecting words with higher probabilities, resulting in more predictable and structured text.
The temperature parameter allows you to find the right balance between creative flair and predictability, depending on your specific text generation goals.
Maximum Length: Controlling Output Size
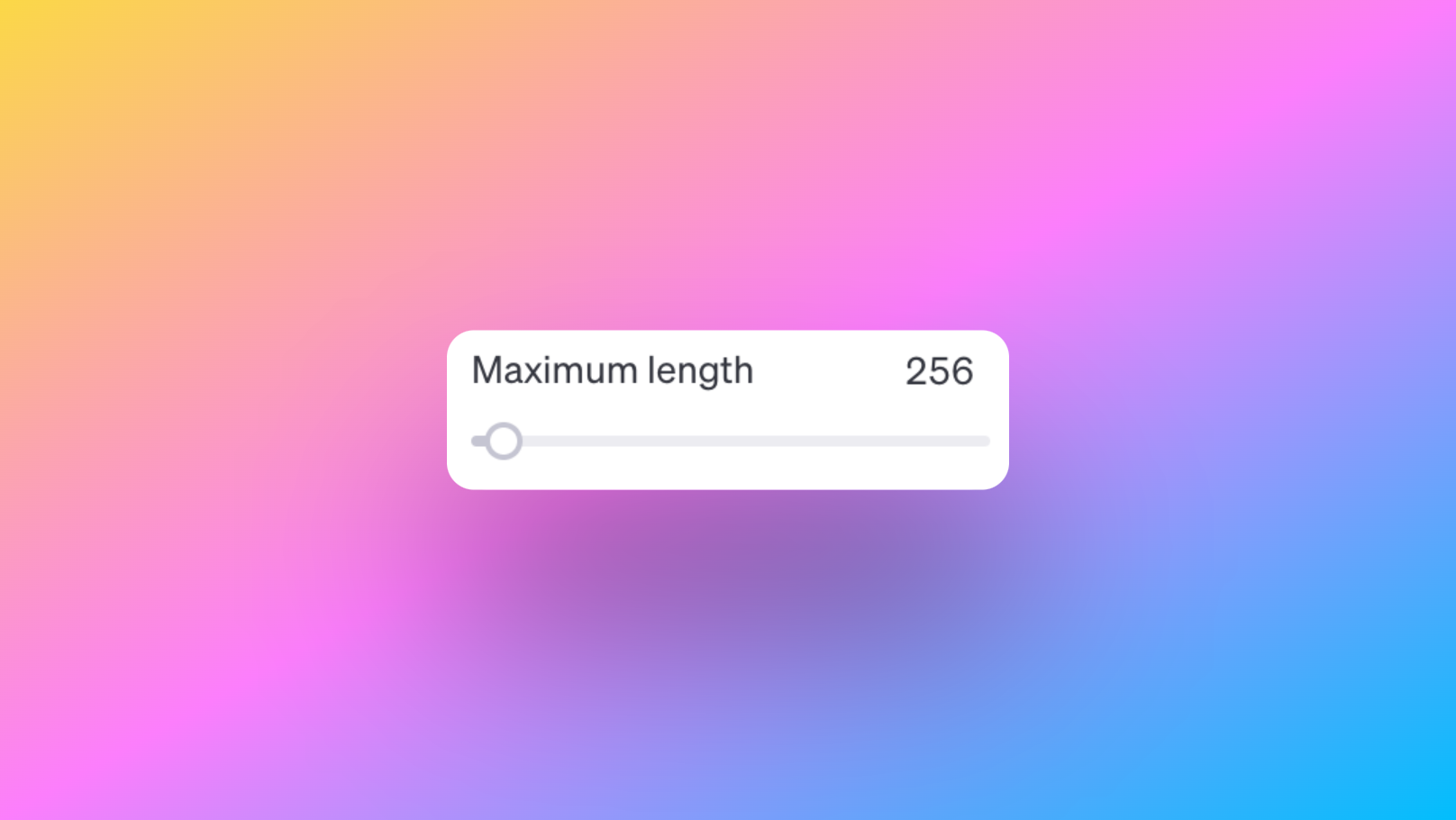
The Maximum Length parameter enables you to control the size of the generated text. You can specify the maximum number of tokens you want in the output. For example, if you set it to 256 tokens, the generated text will not exceed this limit.
This parameter is handy when you need to generate text of a specific length to fit within a character limit or to ensure that the generated content is concise and to the point.
Stop Sequences: Defining End Points
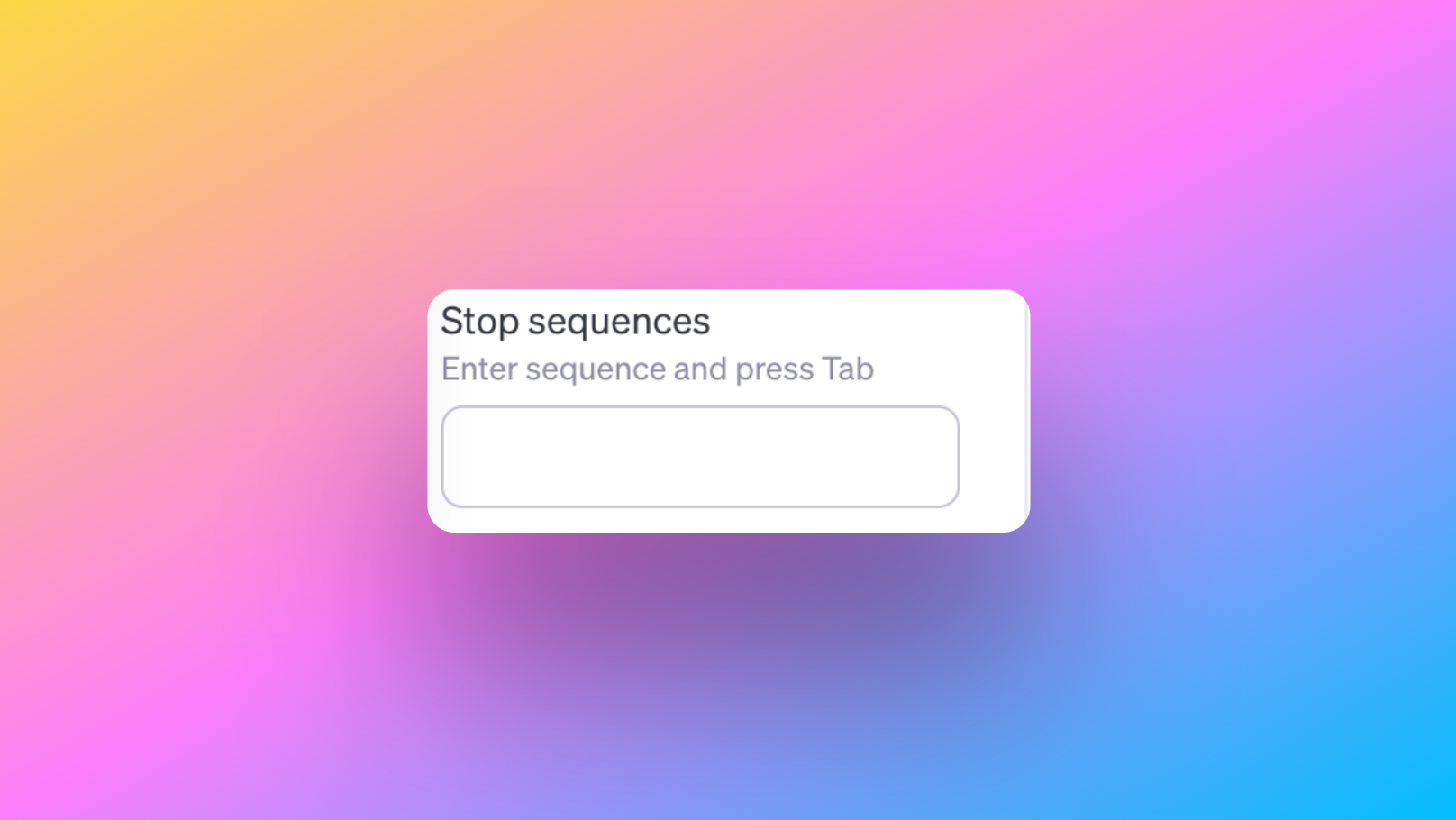
The Stop Sequences parameter is useful when you want to specify certain sequences that indicate the end of the generated text. You can enter a sequence and press Tab to define it as a stop sequence. When the model encounters this sequence in the generated text, it will treat it as the endpoint and stop generating further content.
This feature is valuable when you need precise control over where the generated text should conclude or if you want to ensure that certain information is always present at the end of the generated content.
Frequency Penalty: Encouraging Variety
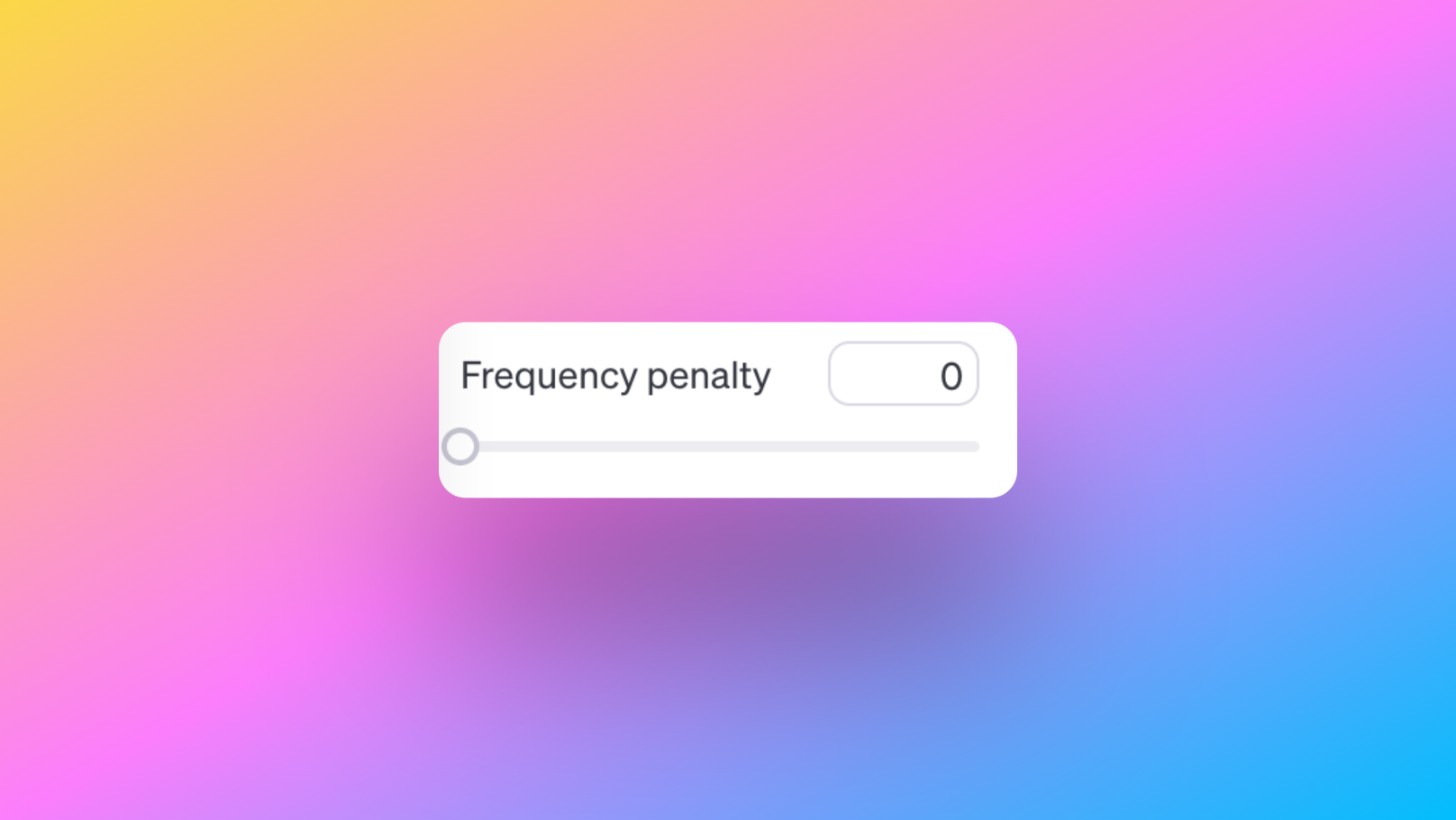
The Frequency Penalty parameter allows you to encourage diversity in the generated text. By increasing the frequency penalty value, you prompt the model to avoid repeating the same words or phrases excessively.
For instance, setting a higher frequency penalty value like 0.8 will make the model less likely to reuse words frequently, resulting in more varied and engaging text.
Presence Penalty: Discouraging Specific Content
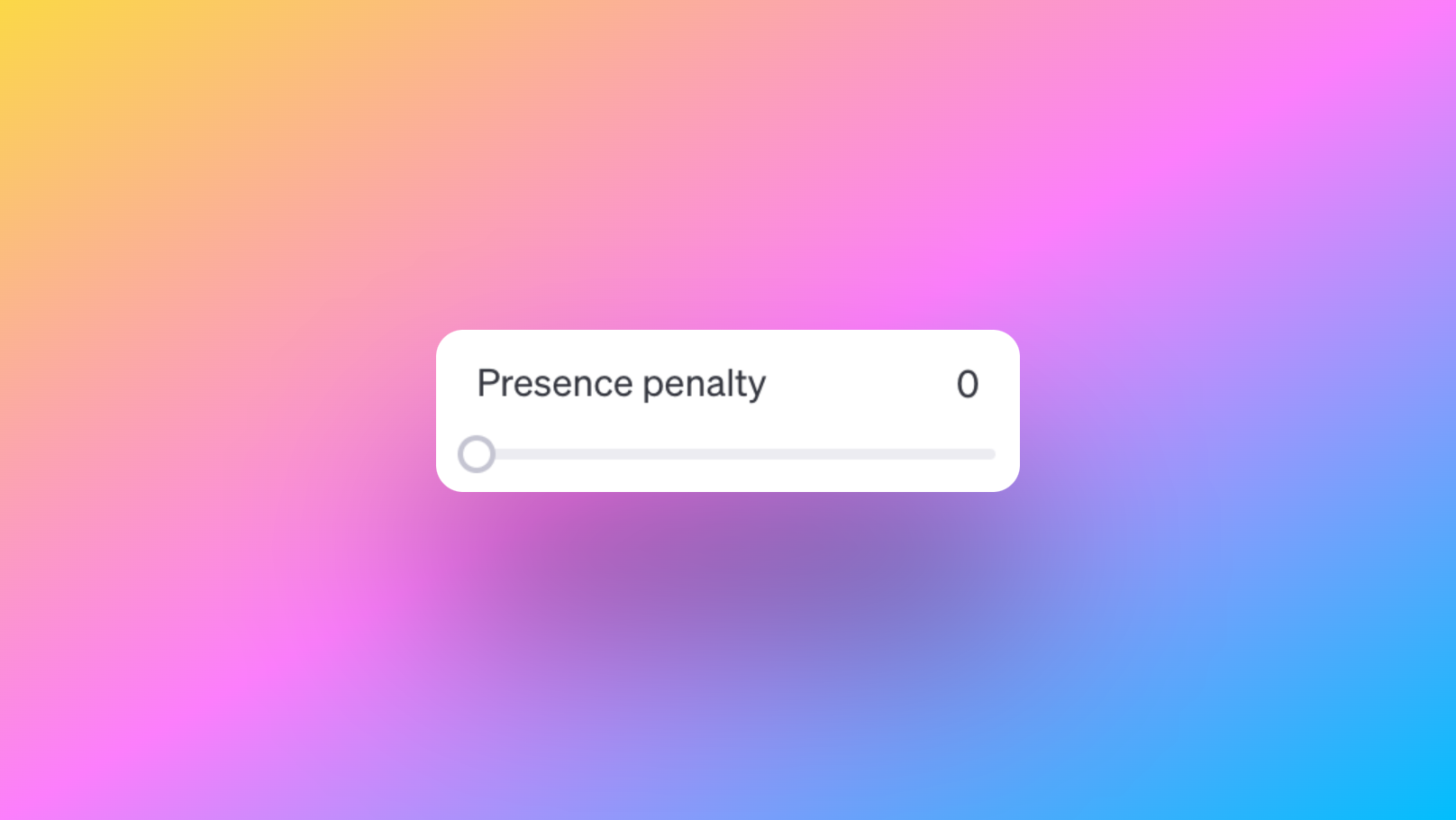
On the flip side, the Presence Penalty parameter is designed to discourage the inclusion of specific content in the generated text. You can increase the presence penalty value to make the model less likely to include certain words or phrases in the output.
For example, setting a high presence penalty value like 0.8 will prompt the model to avoid using specific terms, ensuring that they are less prevalent in the generated content.
Conclusion
In conclusion, the top-p parameter is a pivotal element in fine-tuning text generation in the OpenAI Playground. It empowers users to control the diversity and creativity of generated text while maintaining relevance to the context. By understanding how the top-p parameter functions and how it compares to the top-k parameter, you gain the insights needed to harness the full potential of AI-driven text generation for your specific needs.
Want to test out the ChatGPT powered AI Agent?
Use Anakin AI for creating any AI Agent at your will!
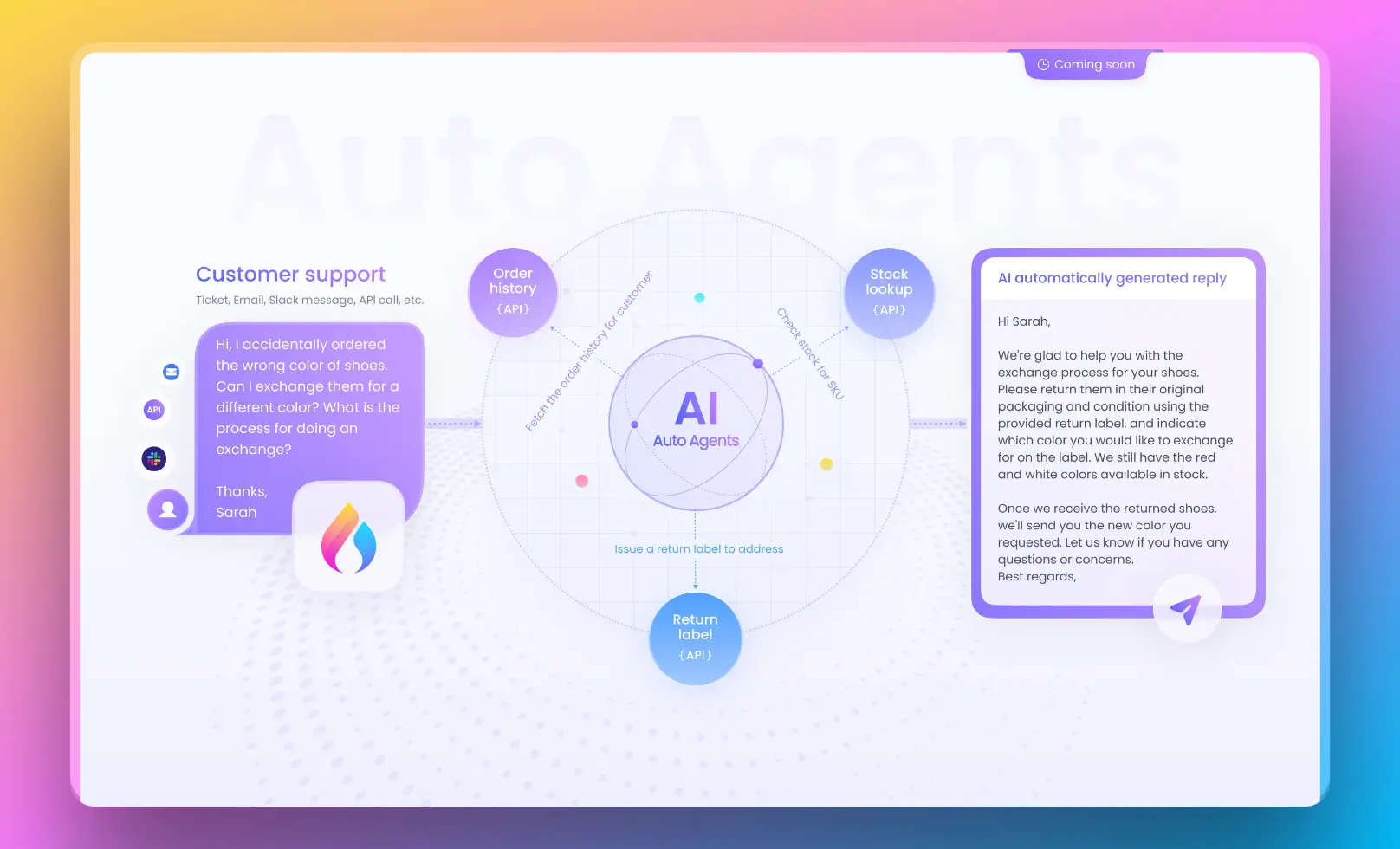