AIランドスケープの注目すべきイベントとして、ミストラルAIのMixtral 8x7Bは大きな進歩として際立っています。このオープンソースの大規模言語モデル(LLM)は、単なる新たな追加ではなく、地元のミニGPT-4に匹敵するものです。
Mixtral 8x7B:GPT-4のコンパクトバージョン
Mixtral 8x7Bは、GPT-4のよりコンパクトで効率的なバージョンであり、より扱いやすくアクセスしやすい形式で高度なAI機能を提供します。
ミストラルAIは、類似の専門家の混合(MoE)アーキテクチャを採用していますが、縮小された形式で、様々なアプリケーションに対する実用的な代替手段となっています。
Mixtral 8x7Bの主な特徴:
- 構造:GPT-4よりも大規模な7,000億のパラメータを持つ専門家8人を利用しています。
- 効率的な処理:推論にはトークンごとに2人の専門家のみを使用し、GPT-4の効率と同様のパフォーマンスを発揮します。
技術的なパラメータ:
- サイズ:モデルは87GBであり、8人のMistral 7Bよりも小さく、サイズ削減のために共有された注意パラメータを示しています。
- GPT-4との比較:専門家の数は2倍に減少し、各専門家あたりのパラメータ数は24倍に減少し、推定される合計パラメータ数は420億です。
コンテキストの容量:
- GPT-4と同様の32Kのコンテキストサイズを維持し、堅牢なパフォーマンスを提供します。
ミストラルAIの新しいLLMドロップについてなぜ誰もが話しているのか?
- Mistral AIによって開発されました:このパリを拠点とするスタートアップは、技術的な専門知識とオープンソースの利便性を組み合わせて注目を集めています。
- AI業界へのインパクト:Mixtral 8x7Bのリリースは、業界の常識に挑戦し、AI技術の民主化を促進するパラダイムシフトを示しています。
- AIの成果に加えて:Mixtral 8x7Bは、AIイノベーションのリストを向上させるだけでなく、既存の基準に挑戦し、イノベーションと探求の新たな道を開くものです。
- オープンでアクセスしやすいAIへの道を開拓する:ミストラルAIのMixtral 8x7BはAI開発におけるオープンさと協力の新たな前例を示しています。
Mixtral 8x7B Torrentのダウンロード
ミストラルAIは、通常の派手なローンチではなく、Mixtral 8x7Bをトレントリンクでリリースするというユニークな道を選びました。
ここに最新のMixtral 87XBモデルのトレントリンクがあります:
magnet:?xt=urn:btih:208b101a0f51514ecf285885a8b0f6fb1a1e4d7d&dn=mistral-7B-v0.1&tr=udp%3A%2F%http://2Ftracker.opentrackr.org%3A1337%2Fannounce&tr=https%3A%2F%https://t.co/HAadNvH1t0%3A443%2Fannounce
RELEASE ab979f50d7d406ab8d0b07d09806c72c
magnet:?xt=urn:btih:5546272da9065eddeb6fcd7ffddeef5b75be79a7&dn=mixtral-8x7b-32kseqlen&tr=udp%3A%2F%http://2Fopentracker.i2p.rocks%3A6969%2Fannounce&tr=http%3A%2F%http://2Ftracker.openbittorrent.com%3A80%2Fannounce
RELEASE a6bbd9affe0c2725c1b7410d66833e24
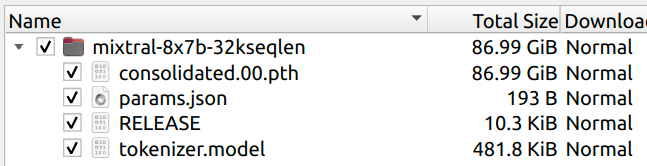
この方法は、GoogleやOpenAIのような企業によるより練り込まれたリリースとは対照的であり、スペクタクルを作り出すよりも声明を出すことに重点を置いています。
この非伝統的なリリースは、モデルの能力だけでなく、AI技術の未来やオープンソースモデルの役割についての議論を引き起こしました。
ミストラル vs グーグル pic.twitter.com/mJSJ252nga
— Manoj Mahalingam (@manojlds) December 8, 2023
コードなしでAIアプリを作成したいですか?
Anakin AIを試してみてください- GPT-4、Claude-2.1、DALLE-3、Stable DiffusionなどのAIモデルを使用してアプリを構築できるNo Codeプラットフォームです!
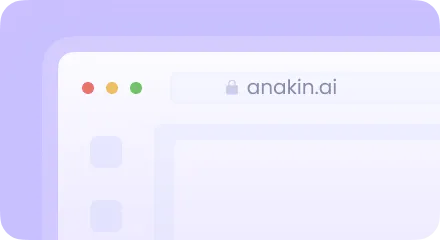
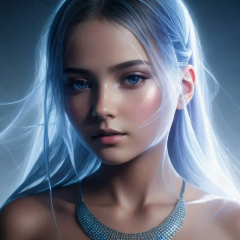
Mixtral 8x7Bの動作原理は?
Mixtral 8x7Bの核心に入ると、モダンなAIエンジニアリングの驚異が見つかります。これは、ミストラルAIの専門知識とビジョンを証明するものであり、広く評価されてきたGPT-4の小型版以上の価値があります。
Mixtral 8x7Bは、効率と拡張性のために近年注目されている専門家の混合モデル(MoE)の原理に基づいて動作します。具体的には、Mixtral 8x7Bは8つの専門家からなり、それぞれが70億のパラメータを持ちます。これはGPT-4の1110億のパラメータを持つ8人の専門家からの大幅なスケールダウンです。この設計の選択肢は、計算要件の管理が容易になるだけでなく、さまざまなアプリケーションに対応できるようになっています。
Mixtral 8x7Bのアーキテクチャは特に魅力的です。トークンの推論には、8人の専門家のうちの2人のみが使用されるように設計され、処理効率と速度を最適化する戦略が採用されています。このアプローチは、モデルのメタデータに反映されており、より深い洞察を提供します:
{
"dim": 4096,
"n_layers": 32,
"head_dim": 128,
"hidden_dim": 14336,
"n_heads": 32,
"n_kv_heads": 8,
"norm_eps": 1e-05,
"vocab_size": 32000,
"moe": {
"num_experts_per_tok": 2,
"num_experts": 8
}
}
この構成は、高次元の埋め込み空間(dim: 4096
)、複数のレイヤー(n_layers: 32
)、および注意メカニズムのための大量のヘッド(n_heads: 32
)を特徴とする高度なセットアップを示しています。
The MoE architecture ("moe": {"num_experts_per_tok": 2, "num_experts": 8}
) underscores the model’s focus on efficient, specialized processing for each token.
One of the most remarkable aspects of Mixtral 8x7B is its ability to deliver high-level AI capabilities while being considerably more accessible than its larger counterparts. This accessibility is not just in terms of usability but also in terms of the computational resources required to run it. The model's reduced size, compared to a hypothetical 8x scale-up of Mistral 7B, is achieved through shared attention parameters, a clever design choice that significantly reduces the overall model size without compromising its performance.
The implementation of Mixtral 8x7B in practical applications is also noteworthy. The model's design allows for flexible adaptation to various computing environments, from high-end servers to more modest setups. Here's a glimpse into how Mixtral 8x7B can be implemented:
python example_text_completion.py path/to/mixtral/ path/to/mixtral/tokenizer.model
In this simple command, example_text_completion.py
represents a script utilizing the Mixtral 8x7B model, with the paths directing to the model and tokenizer files. This level of straightforward implementation speaks to Mistral AI's commitment to making advanced AI technology more accessible and user-friendly.
You can visit the Mixtral 8x7B Hugging Face page by someone here:
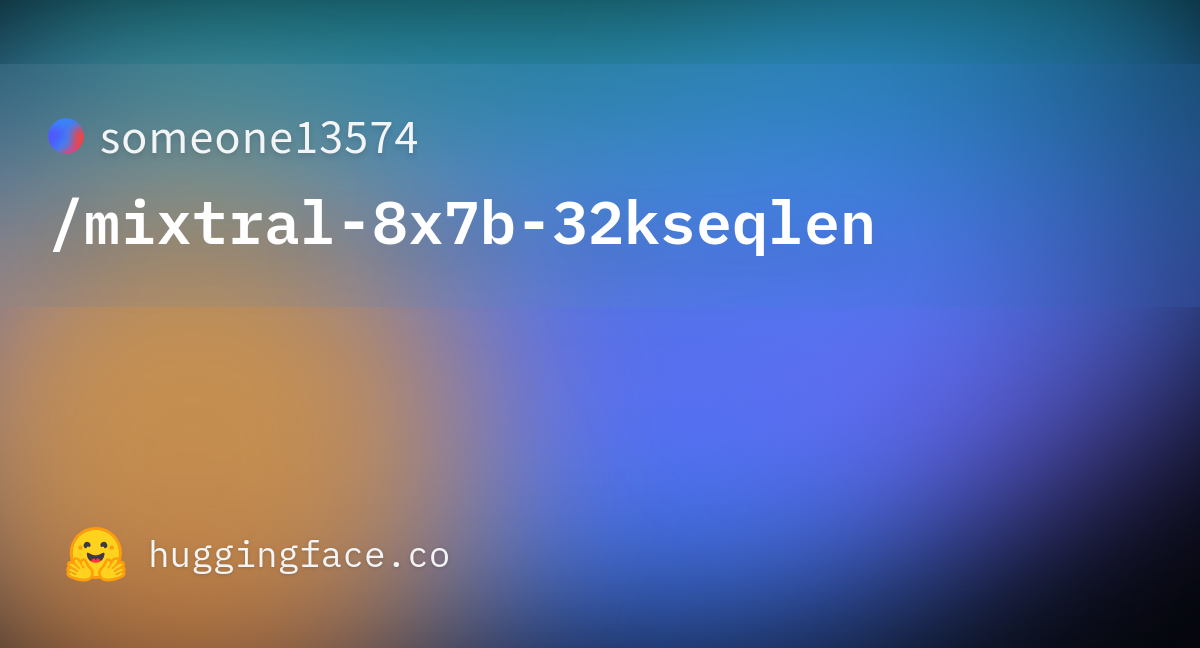
Mixtral 8x7B stands as a powerful yet approachable tool in the AI landscape. Its technical sophistication, combined with its pragmatic design, makes it not only a formidable LLM but also a beacon of what the future of AI models could look like - powerful, efficient, and within reach of a broader range of users and applications.
How to Run Mixtral 8x7B - Two Current Methods
Method 1: Run Mixtral 8x7B with This Hack
For those eager to experiment with Mixtral 8x7B immediately, a somewhat unorthodox but functional method exists. This approach involves adapting the original Llama codebase to work with Mixtral 8x7B, although it's important to note that this implementation is still in its early stages and might not fully leverage the model's capabilities.
Key Points of the Hack:
Naive and Slow: The implementation is admittedly basic and not optimized for speed.
- Hardware Requirements: To run this version, you will need either two 80GB GPUs or four 40GB GPUs.
- Technical Details: The method involves removing model parallelism for simplicity and sharding experts across the available GPUs. It also includes a reverse-engineered MoE implementation based on this research paper.
How to Implement:
Download the Weights: The Mixtral model weights are available either via Torrent or from the Hugging Face repository at Mixtral 8x7B on Hugging Face.
Running the Model: Once you have the weights, you can run the model on 2 GPUs (requiring about 45GB each) using the following command:
python example_text_completion.py path/to/mixtral/ path/to/mixtral/tokenizer.model
For a 4 GPU setup, add --num-gpus 4
to the command.
Method 2. Use Fireworks.ai API to Run Mixtral 8x7B
An alternative, more user-friendly way to test Mixtral 8x7B is through the Fireworks.ai platform. This platform offers a streamlined experience, though it's important to note that this is not an official implementation from Mistral AI.
Key Features of Fireworks.ai:
- Quick Access: The model is available for use just hours after the initial release of the weights, demonstrating the agility and collaborative spirit of the AI community.
- Reverse-Engineered Architecture: The implementation on Fireworks.ai was achieved by reverse-engineering the architecture from the parameter names in collaboration with the community.
Using Fireworks.ai: - Accessing the Model: Simply visit Fireworks.ai to try out Mixtral 8x7B.
- Caveat: Users should be aware that since this is not an official release, the results might vary, and updates are expected once Mistral AI releases the official model code.
Mistral AI: the Best AI Company in the Town
The release of Mixtral 8x7B has rippled across the AI community, eliciting a range of reactions that underscore its significance. Enthusiasts, experts, and developers have all weighed in, highlighting the model's impact from multiple angles.
Positive Buzz and Constructive Criticism: The response has been largely positive, with many praising Mistral AI for their innovative approach and the model's technical capabilities.
Uri Eliabayev, an AI consultant, lauded Mistral for their daring release strategy
George Hotz, a well-known entrepreneur, expressed his appreciation for Mistral’s brand, which he considers a favorite in the AI space.
Google put out a press release and a fake demo.
— George Hotz 🌑 (@realGeorgeHotz) December 8, 2023
Mistral put out a torrent. https://t.co/K3qKWLWQBE
This enthusiasm is tempered by constructive criticism, mainly focused on the model's limitations compared to larger counterparts like GPT-4, but even these critiques acknowledge the potential of Mixtral 8x7B.
Perhaps most importantly, the AI community has shown a strong appreciation for Mistral AI’s commitment to open-source principles. This approach has been hailed as a step towards democratizing AI technology, making it more accessible to a wider range of users and developers.
Mistral AI: Setting New Standards for Open Source LLMs with Mixtral 8x7B
As we look to the future, Mixtral 8x7B’s influence on the AI landscape becomes increasingly apparent. Mistral AI’s approach to releasing and designing Mixtral 8x7B could set new standards for AI model development and dissemination.
Mixtral 8x7B represents a significant push towards more open-source AI solutions.
その成功は、他の企業に同様のアプローチを採用することを促し、より多様で革新的なAI研究環境の形成につながる可能性があります。
Mistral AIのMixtral 8x7Bは、AIとLLM開発の未来をどのように変えるのか
Mixtral 8x7Bへの熱烈な支持とそれによって引き起こされた議論は、よりオープンで協力的なAI開発への成長するトレンドを示しています。このモデルは、さまざまなアプリケーションにとって貴重なツールを提供するだけでなく、AIフィールドでの革新、探求、議論の新たな可能性を開拓しました。
今後、Mixtral 8x7BはAIの未来を形作る上で重要な役割を果たすでしょう。その影響は、産業やコミュニティ全体に波及し、さらなる進展をもたらし、最先端のAI技術へのアクセスを民主化するでしょう。要約すると、Mixtral 8x7Bは単にMistral AIにとっての一つの里程碑に過ぎず、AI開発において包括的で協力的かつオープンな方向への転換を示しています。
No CodeでAIアプリを作成に興味がありますか?
Anakin AIを試してみてください。GPT-4、Claude-2.1、DALLE-3、Stable DiffusionなどのAIモデルを使用してアプリを構築できます!
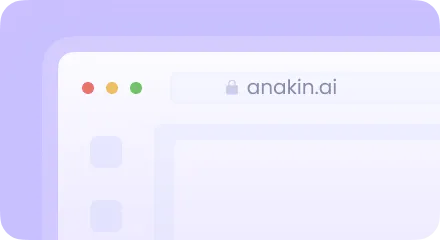
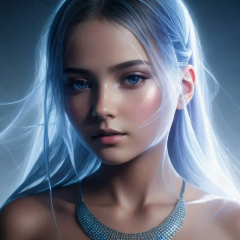