The magic of stable diffusion lies in its ability to create detailed and realistic images, sometimes indistinguishable from those taken by a camera or drawn by a human hand. However, the quality and accuracy of these images heavily depend on the sampling method you used for Stable Diffusion. This is where choosing the right sampling method becomes crucial. Different methods can lead to vastly different results in terms of image clarity, realism, and relevance to the input prompt.
Key Takeaways: Summarizing the Important Points
- Diverse Methods: There's a wide range of samplers available, each suited for different needs.
- Balancing Act: The choice of Stable Diffusion sampling methods often involves balancing between speed, detail, and control.
- Continual Experimentation: The field of Stable Diffusion art is evolving, and staying open to experimenting new tools, such as using Stable Diffusion Image Generator online.
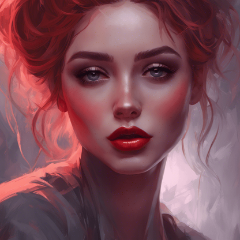
What are 'Sampling Steps' in Stable Diffusion, Anyway?
In stable diffusion, sampling methods are algorithms that guide how an AI transforms random noise into a coherent image. Think of it like a painter who starts with a blank canvas and gradually adds layers of paint to create a picture. These methods dictate how each 'brushstroke' is applied, influencing the final appearance, detail, and accuracy of the image.
'Sampling steps' in stable diffusion are like the individual brushstrokes in our painting analogy. Each step is a phase where the AI makes adjustments to the image, getting it closer to the final result. Fewer steps mean a faster process but can result in less detail. More steps allow for finer details but take longer. The key is finding the right balance for the desired outcome.
Basics Types of Sampling in Stable Diffusion
DPM++ SDE Karras
DPM++ SDE Karras is one of the key sampling methods used in stable diffusion.
- DPM++ SDE Karras stands for Deep Probabilistic Modeling with Stochastic Differential Equations, a method developed by Timo Karras and his team.
- This method is known for its efficiency and effectiveness in generating high-quality images.
DPM++ 2M Karras
Taking a closer look at DPM++ 2M Karras, this method stands out for its advanced approach to handling the diffusion process.
- DPM++ 2M Karras incorporates a mixture of techniques that enhance the image's realism and detail while maintaining a reasonable generation speed.
- DPM++ 2M Karras can turn complex AI algorithms and models, but its application results are evident in the strikingly realistic and detailed images it produces.
What is the Difference Between DPM++ SDE Karras And DPM++ 2M Karras?
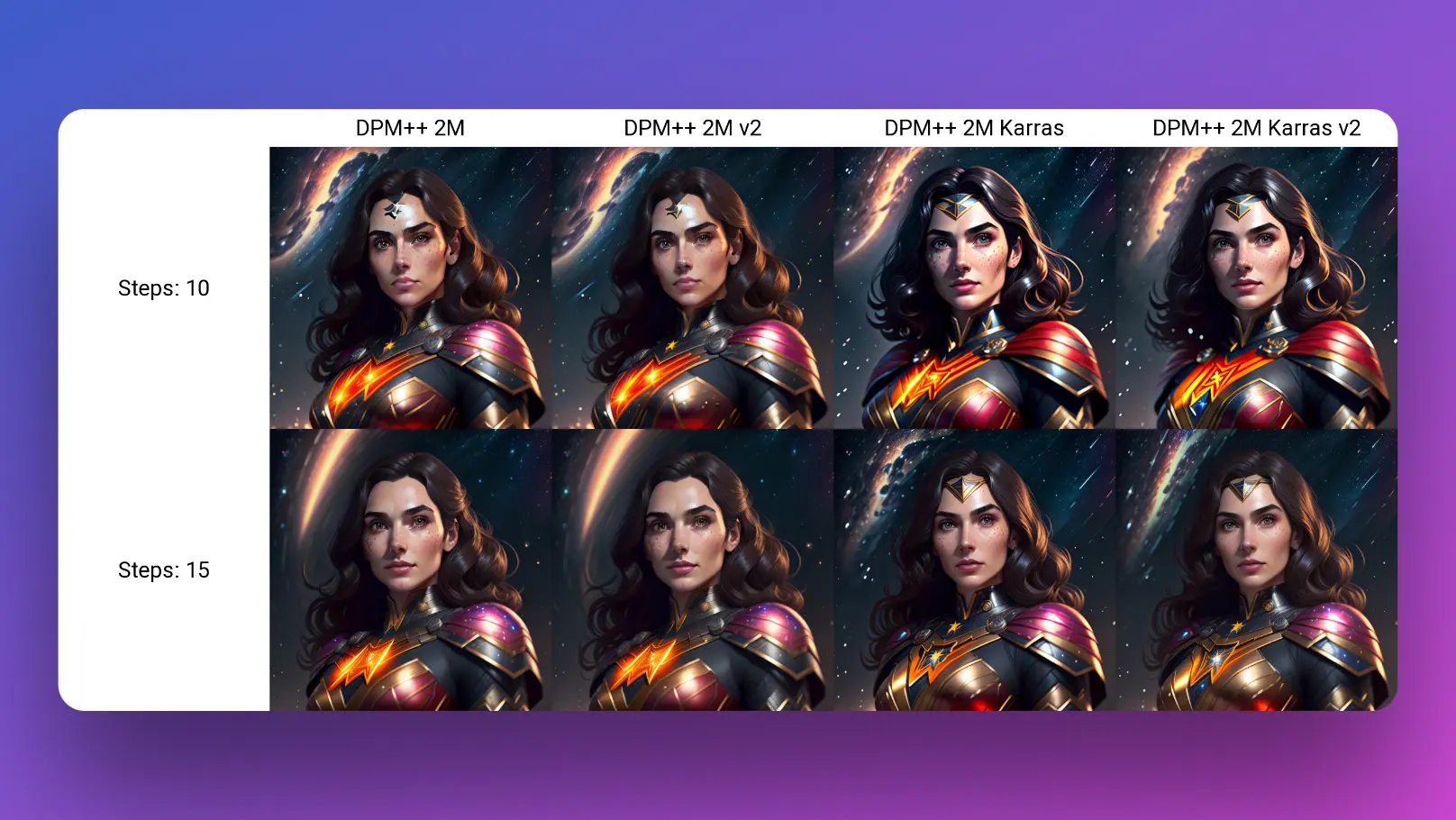
In a side-by-side comparison, different sampling methods reveal their strengths and weaknesses. For instance, DPM++ 2M excels in generating detailed and complex images quickly, while Euler A provides a balance between speed and image quality. DDIM (Denoising Diffusion Implicit Models), on the other hand, stands out for its ability to generate images with a high degree of control over the generation process.
Euler A
- Euler A: Known for its balance in speed and quality, ideal for a wide range of applications.
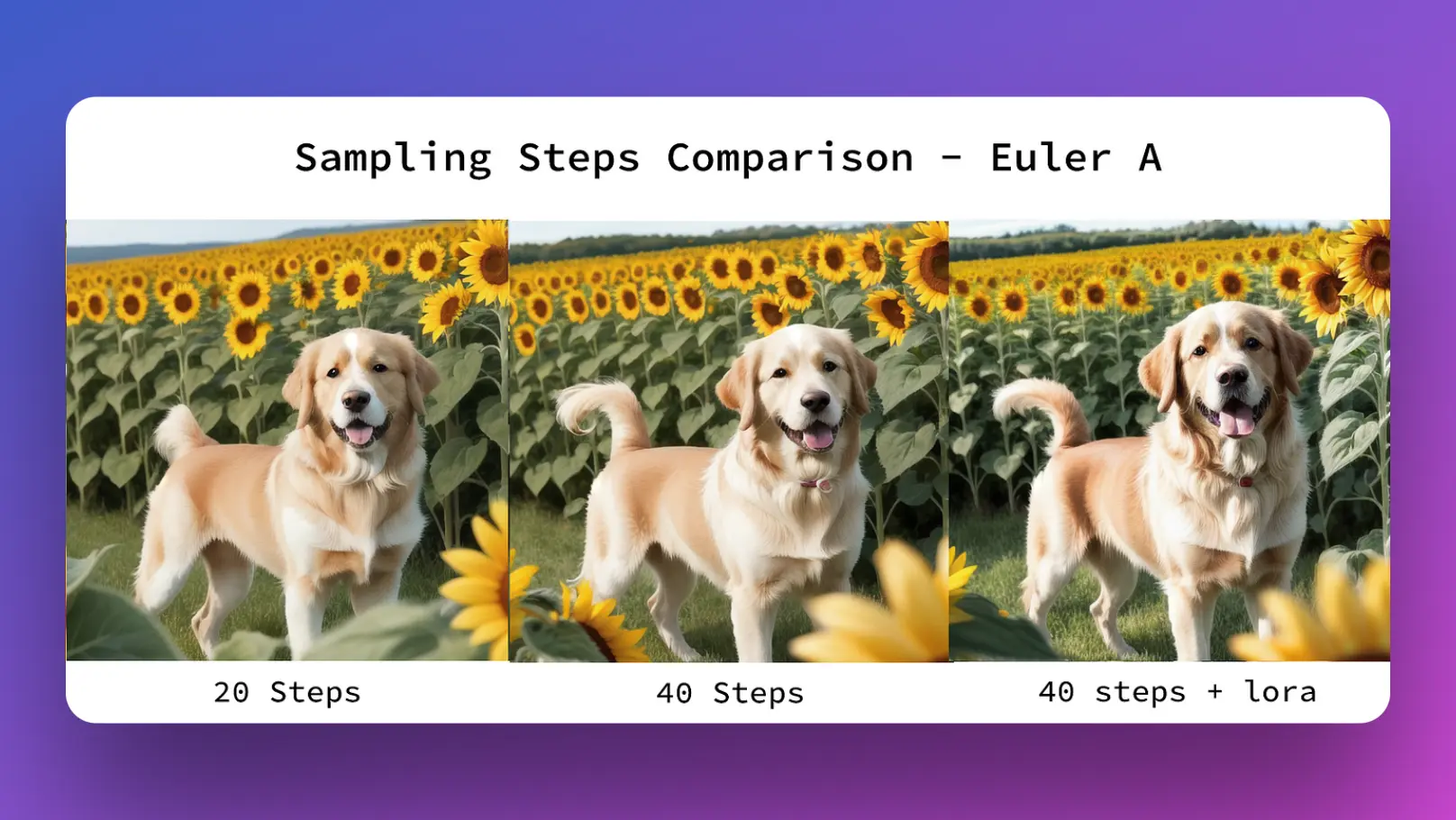
Euler vs. Euler A: What's the Difference?
While both Euler and Euler A are based on the Euler method for solving differential equations, Euler A introduces adaptive adjustments that optimize the sampling process. This results in faster image generation without a significant compromise in image quality, making Euler A a preferred choice in scenarios where speed is a critical factor.
VETS Sampling
VETS, or Variational Energy-based Trajectory Sampling, is another notable method in stable diffusion.
- VETS focuses on optimizing the energy consumption during the sampling process, leading to more efficient image generation.
- VETS can produce high-quality images while minimizing the computational resources required, making it a valuable method for applications where resource efficiency is key.
Other Stable Diffusion Sampling Methods
- DPM Adaptive: Adapts the sampling process based on the complexity of the prompt, offering efficiency in generating complex images.
- DDIM: Offers greater control over the diffusion process, allowing for more precise image generation.
Comparing Different Samplers in Stable Diffusion
In stable diffusion, samplers are algorithms that guide the AI in generating images from noise. The comparison image showcases various samplers and the results they produce, demonstrating that each sampler has its own style and capabilities.
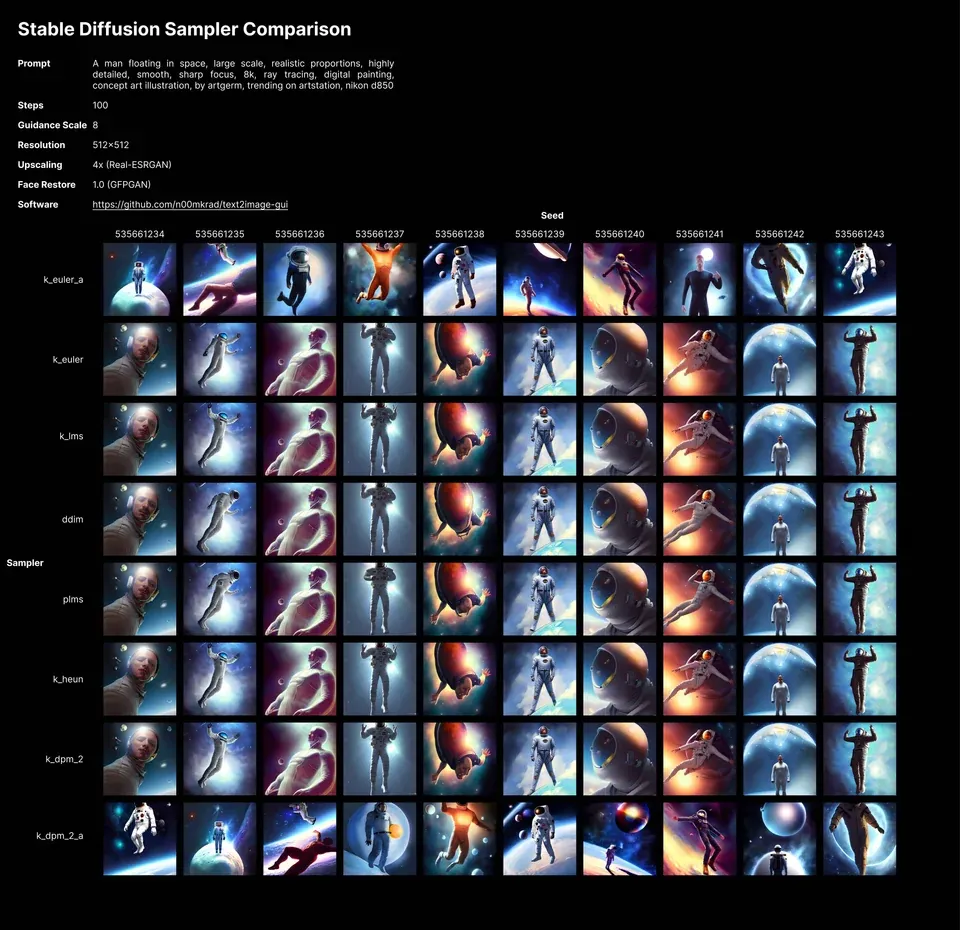
Let's discuss the characteristics and use cases for different samplers as observed:
- K_euler and K_euler_a: These samplers appear to maintain a balance between image fidelity and artistic interpretation. They are suitable for scenarios where you want a blend of realism with a touch of artistic flair.
- K_lms: The images generated with this sampler exhibit a high degree of clarity and detail, making K_lms a good choice for projects where precision is valued.
- Ddim: This sampler seems to offer images with a smoother texture. Its control over the diffusion process makes it ideal for users who need more influence on the outcome.
- Plms: The images here show a unique texture and style. This method might be preferred in creative endeavors where a distinctive look is desired.
- K_heun: The visuals produced with this sampler suggest a more experimental approach, possibly beneficial for abstract or conceptual art.
- K_dpm_2 and K_dpm_2_a: These samplers demonstrate a noticeable variance in lighting and texture, hinting at their utility for dynamic and vivid image generation.
Which Sampling Method Shall I Use?
In a side-by-side comparison, different sampling methods reveal their strengths and weaknesses. You can select the best sampler based on your specific needs:
- For High Detail: If the goal is intricate detail, like in portraits or landscapes, go for a method like DPM++ 2M or LMS Karras.
- For Speed: When speed is a priority, Euler A or DPM Adaptive are great choices.
- For Control: If you need more control over the image outcome, DDIM provides a more hands-on approach.
Are more sampling steps better in Stable Diffusion?
Not necessarily.
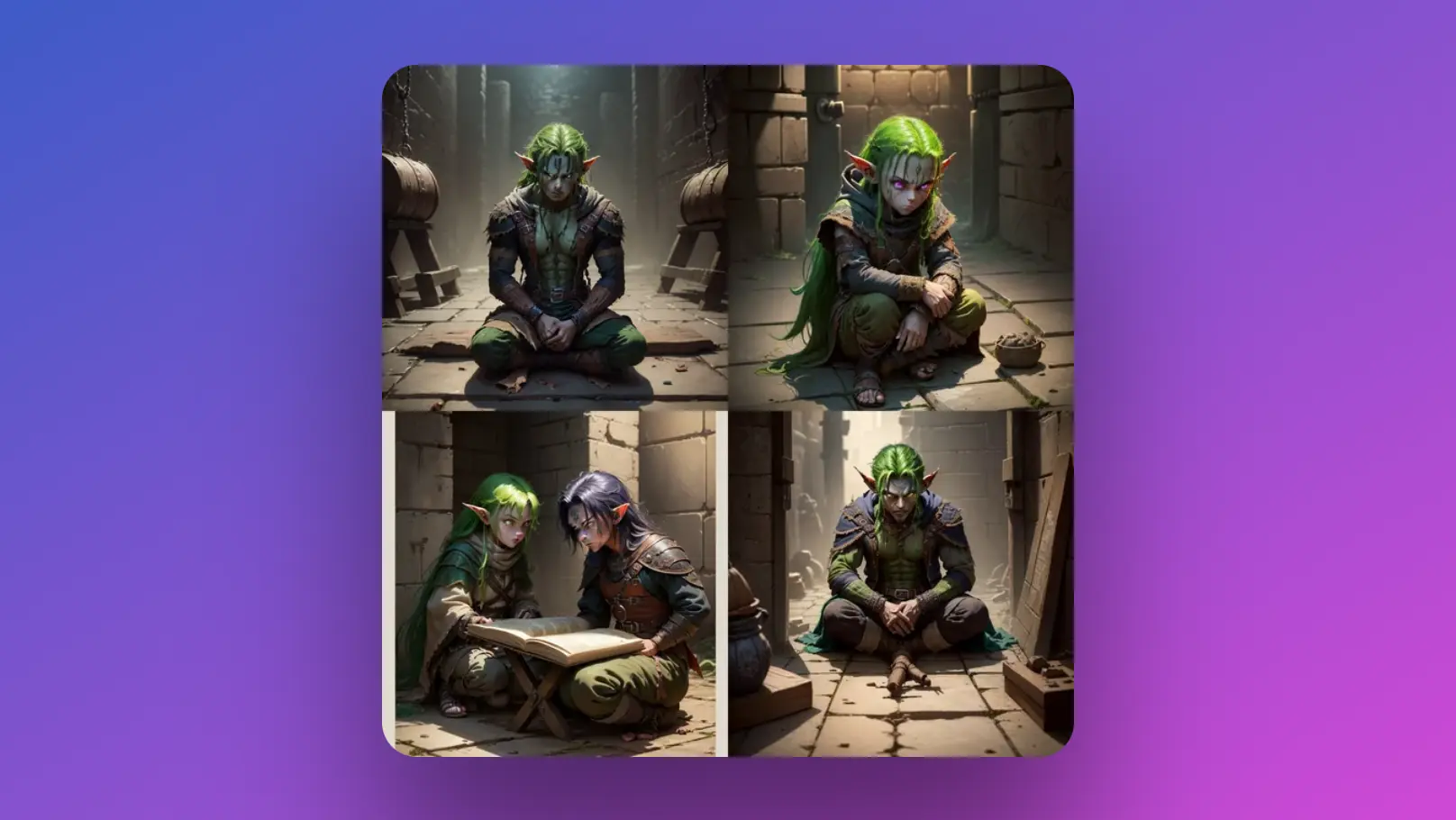
More sampling steps typically lead to a more refined and detailed image, as the AI has more opportunities to adjust and hone the picture. This can be crucial for creating highly detailed or realistic images.
However, more steps also mean a longer processing time, without a significant quality improvement that you can visually see on the screen. In many cases, a moderate number of steps can provide a good compromise between image quality and generation speed.
One benefit of having more sampling steps is that: You can generate more realistic images with Stable Diffusion.
Want to test out the features of Stable Diffusion Online?
Try Stable Diffusion Online Image Generator Now!
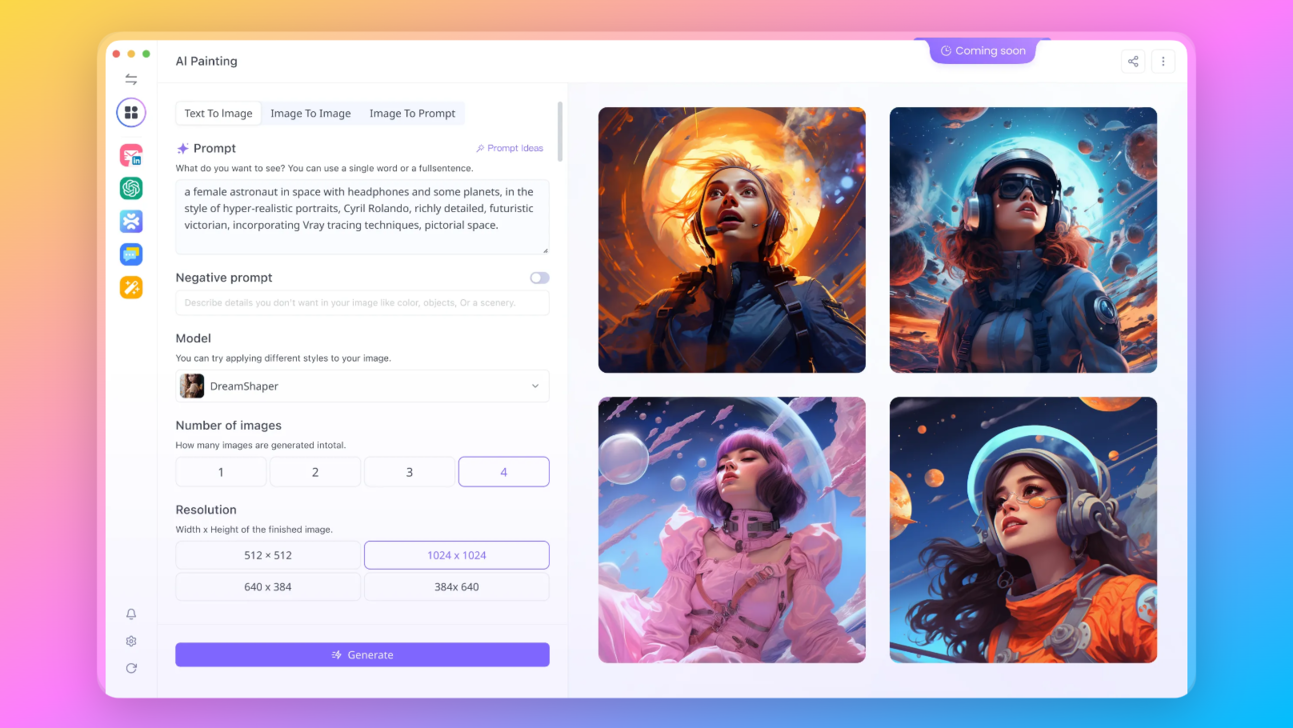
What is CFG Scale in Stable Diffusion?
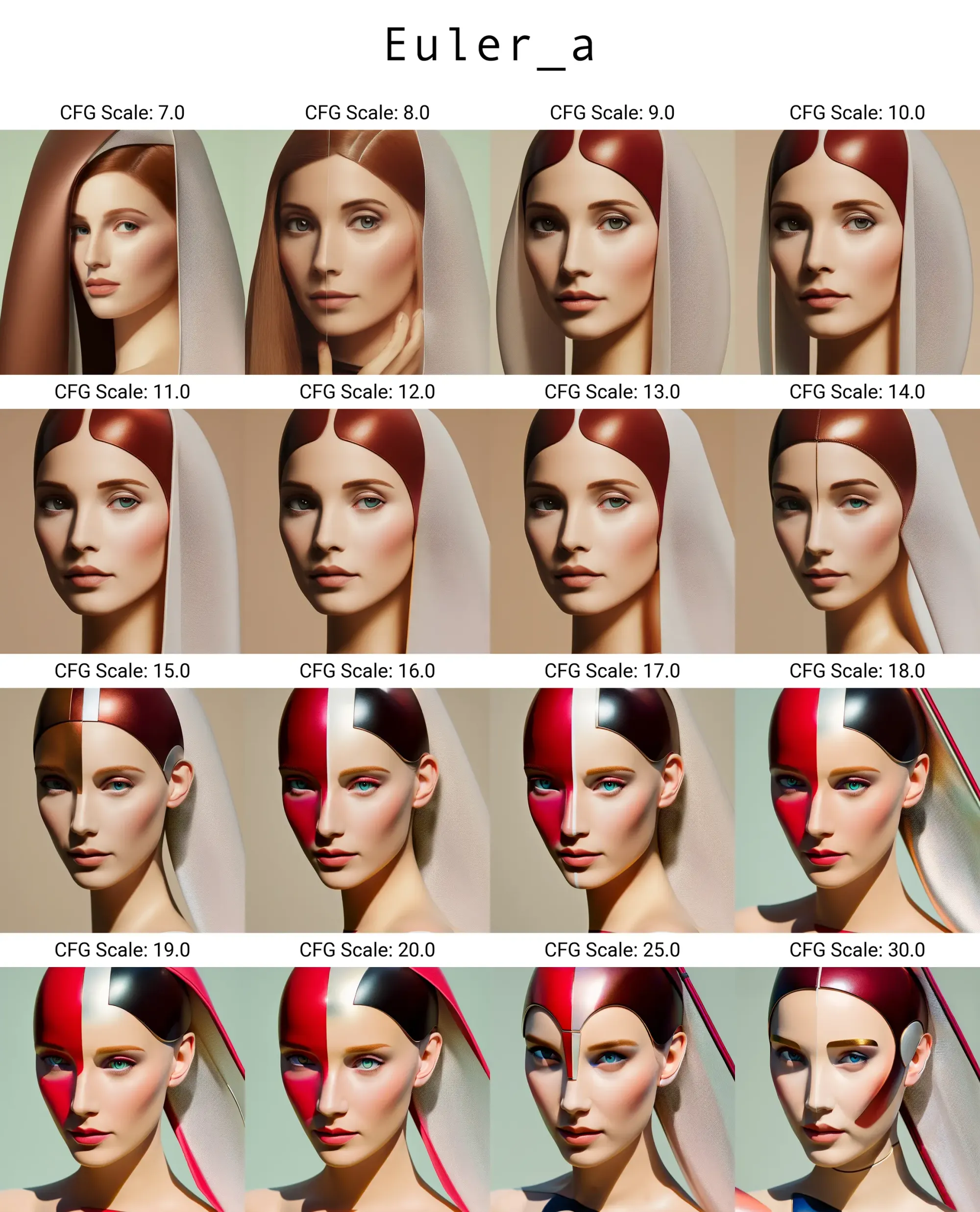
The CFG scale, or "Classifier Free Guidance" scale, is an essential concept in the realm of stable diffusion. This scale is used to control the level of adherence of the generated image to the input prompt. Essentially, the CFG scale acts as a tuning knob that adjusts how closely the AI-generated image follows the specific details and instructions provided in the user's prompt.
- At lower CFG scale values, the AI has more creative freedom, which means it can deviate more from the exact details of the prompt, leading to more abstract or stylized images.
- Conversely, higher CFG scale values tighten the adherence to the prompt, resulting in images that more accurately reflect the specified details
Want to test out the features of Stable Diffusion Online?
Try Stable Diffusion Online Image Generator Now!
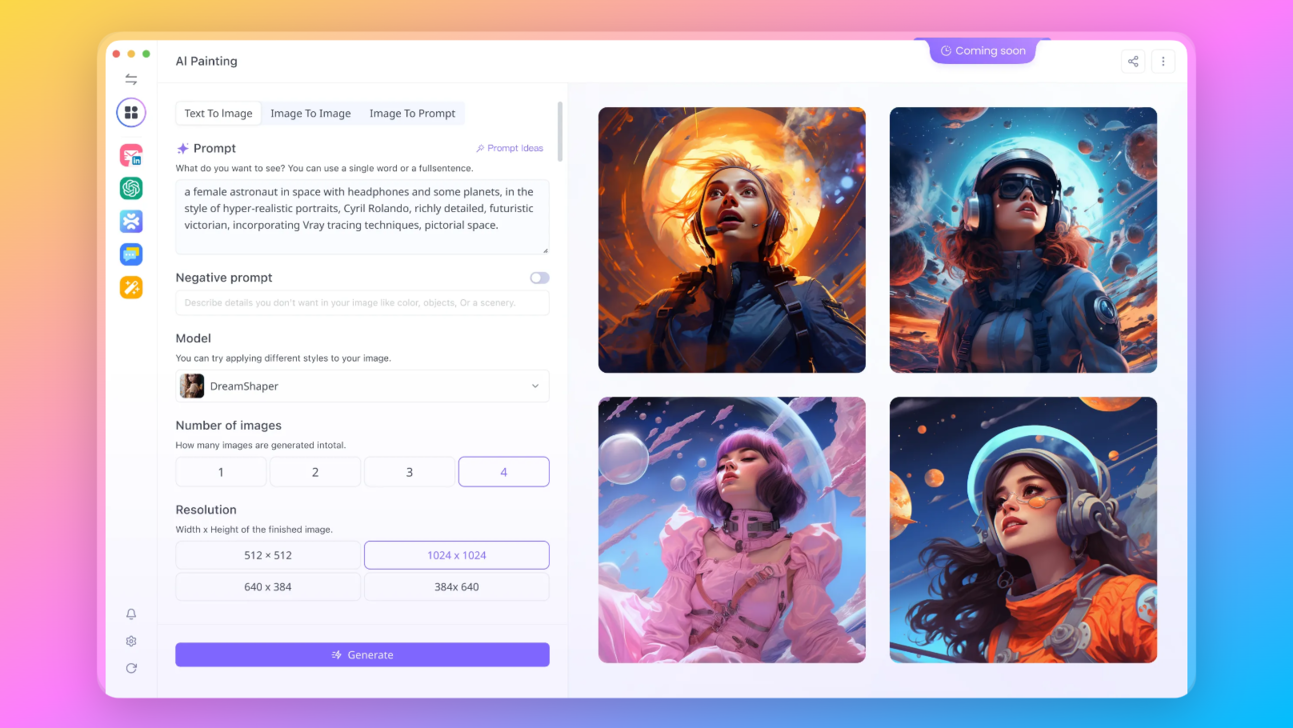
Conclusion
In summary, understanding the technical aspects of stable diffusion sampling methods and choosing the right one can greatly influence the quality and efficiency of the AI-generated images. Whether prioritizing detail, speed, or control, there's a sampler tailored to every need.