In the fast-paced world of artificial intelligence, breakthroughs and innovations are announced almost daily. However, not all claims live up to their hype. The recent release of Reflection 70B, touted as a revolutionary open-source language model, has sparked intense debate within the AI community. Is it truly a game-changing advancement, or just another case of overblown marketing? Let's dive deep into the controversy surrounding Reflection 70B and attempt to uncover the truth.
Searching for an AI Platform that gives you access to any AI Model with an All-in-One price tag?
Then, You cannot miss out Anakin AI!
Anakin AI is an all-in-one platform for all your workflow automation, create powerful AI App with an easy-to-use No Code App Builder, with Llama 3, Claude, GPT-4, Uncensored LLMs, Stable Diffusion...
Build Your Dream AI App within minutes, not weeks with Anakin AI!
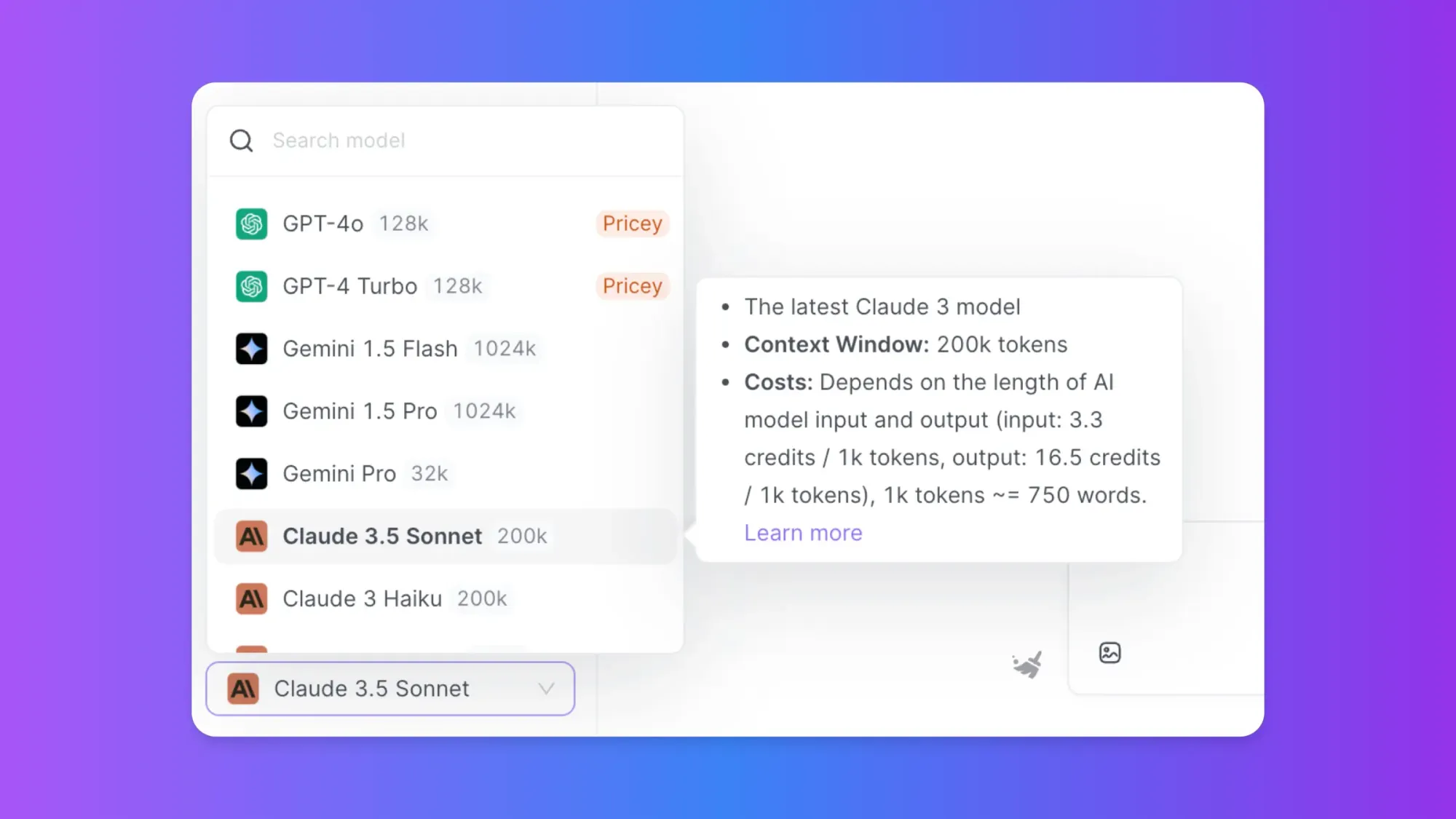
The Initial Announcement of Reflection 70B
Reflection 70B burst onto the scene with bold claims from its creator, Matt Shumer. According to Shumer, this new model was based on Meta's Llama 3.1 70B and incorporated innovative "reflection" techniques to dramatically improve its performance. The hype reached fever pitch when Shumer declared that Reflection 70B outperformed even top closed-source models like Claude 3.5 Sonnet and GPT-4 on various benchmarks.
These extraordinary claims naturally drew significant attention from AI researchers, developers, and enthusiasts. The prospect of an open-source model rivaling or surpassing the capabilities of proprietary giants was tantalizing. However, as the community began to dig deeper, cracks in the narrative started to appear.
Red Flags and Inconsistencies
Benchmark Discrepancies
One of the first issues to arise was the inability of independent researchers to replicate the benchmark results claimed by Shumer. Multiple attempts to evaluate Reflection 70B against standard metrics showed performance that was not only below the stated figures but often worse than the base Llama 3.1 70B model it was supposedly built upon.
This discrepancy raised serious questions about the validity of the original claims. Were the initial benchmarks somehow flawed or manipulated? Or was there a problem with the model weights made available to the public?
Technical Inconsistencies
As the AI community began to examine the model more closely, several technical red flags emerged:
Model Confusion: There was uncertainty about which exact version of Llama the model was based on. While claimed to be built on Llama 3.1 70B, analysis suggested it might actually be using Llama 3 70B instead.
Unusual File Structure: The model weights were initially split into an unusually large number of files, making it difficult to download and work with. This is atypical for well-organized model releases.
Dtype Issues: There was confusion surrounding the data type used in the model, another basic detail that should be clear in a properly documented release.
API Inconsistencies: Users reported significant quality differences between results from the hosted API and locally run versions of the model.
These technical issues compounded the growing skepticism surrounding Reflection 70B. For many in the AI community, they were reminiscent of amateurish mistakes rather than what one would expect from a groundbreaking model release.
The Reflection Technique
At the core of Reflection 70B's purported advancements was the novel "reflection" technique. This approach involved fine-tuning the model on a dataset enhanced with special tokens like , , and . The idea was to encourage the model to use chain-of-thought reasoning and self-correction mechanisms.
While the concept itself is interesting and builds on established ideas in AI research, questions arose about its true effectiveness:
Benchmark Tuning: Some researchers speculated that the model might have been overfitted to specific benchmarks rather than genuinely improving general reasoning capabilities.
Token Overhead: The reflection process adds significant overhead in terms of token usage, potentially making the model less efficient for real-world applications.
Limited Scope: The effectiveness of self-correction is limited to cases where the model realizes it has made a mistake, which may not cover all error scenarios.
Community Response and Investigation
As doubts grew, the AI community on platforms like Reddit began to investigate more thoroughly. Users in subreddits like r/LocalLLaMA and r/singularity shared their experiences, test results, and analyses.
Independent Evaluations
Several users attempted to run Reflection 70B through various tests and real-world scenarios. The results were largely disappointing:
- Many reported that the model performed worse than the base Llama 3.1 70B on practical tasks.
- Coding ability was found to be subpar compared to other available models.
- The 8K context limit was seen as a significant drawback for a model claiming to be state-of-the-art.
Technical Analysis
More technically inclined members of the community dug into the model's architecture and weights:
- Comparisons of the model weights suggested that Reflection 70B might actually be Llama 3 with some LoRA (Low-Rank Adaptation) tuning applied, rather than a more comprehensive fine-tuning of Llama 3.1.
- The reflection technique, while interesting, was found to be less revolutionary than initially claimed. Similar approaches have been used by other models and researchers before.
The Creator's Response
As criticism mounted, Matt Shumer attempted to address the concerns raised by the community. His responses included:
- Claiming that there were issues with the hosted APIs and that the internal version was performing better.
- Promising updates and fixes to the model weights on Hugging Face.
- Suggesting that the community might not be using the correct system prompt to achieve optimal results.
However, these explanations did little to quell the growing skepticism. Many in the community felt that the constant promises of updates and fixes were more indicative of a rushed, flawed release rather than a genuinely groundbreaking model.
Ethical Concerns and Transparency Issues
Beyond the technical aspects, the Reflection 70B controversy raised important ethical questions:
Disclosure of Interests
It came to light that Matt Shumer had not initially disclosed his investment in GlaiveAI, a company involved in the development of Reflection 70B. This lack of transparency raised concerns about potential conflicts of interest and the objectivity of the claims made about the model's performance.
Responsibility in AI Announcements
The incident highlighted the need for responsible communication in AI research and development. Overhyping capabilities or making unsubstantiated claims can erode trust in the field and potentially mislead both the public and other researchers.
Lessons for the AI Community
The Reflection 70B controversy serves as a cautionary tale for the AI community:
Skepticism is Healthy: The initial excitement around Reflection 70B was understandable, but the community's subsequent skepticism and thorough investigation were crucial in uncovering the truth.
Reproducibility is Key: The inability to reproduce claimed results is a major red flag in any scientific or technical field. This incident reinforces the importance of independent verification.
Transparency Matters: Full disclosure of affiliations, methodologies, and limitations is essential for maintaining trust in AI research and development.
Hype vs. Reality: The AI field is prone to hype cycles. It's important for researchers, developers, and the public to maintain a balanced perspective and demand concrete evidence for extraordinary claims.
The Verdict: Real or Scam?
Based on the available evidence and the community's investigations, it's difficult to classify Reflection 70B as a straightforward "scam." However, it's clear that the model fell far short of its initial claims and was plagued by numerous issues:
- Performance did not match the stated benchmarks.
- Technical inconsistencies raised doubts about the model's development process.
- Lack of transparency regarding affiliations and methodology eroded trust.
While the core idea of incorporating reflection techniques into language models may have merit, the execution and communication surrounding Reflection 70B were deeply flawed. At best, it represents a premature and overhyped release. At worst, it could be seen as a misleading attempt to generate buzz and potentially drive investment.
Looking Forward
The Reflection 70B controversy serves as an important moment for the AI community to reflect on its own practices and standards. As the field continues to advance at a rapid pace, it's crucial to maintain:
- Rigorous peer review and independent verification of claims.
- Clear and honest communication about model capabilities and limitations.
- Transparency regarding affiliations, funding, and potential conflicts of interest.
- A healthy skepticism towards extraordinary claims, balanced with openness to genuine innovation.
By learning from incidents like this, the AI community can work towards a more trustworthy and genuinely innovative future. While Reflection 70B may not have lived up to its promises, the discussions and investigations it sparked can contribute to better practices and more reliable advancements in the field of artificial intelligence.