Stable Diffusion has transformed the field of AI art generation by allowing artists and creators to produce stunning and unique artworks. One of the key components of Stable Diffusion is the use of LoRA models, which offer significant advantages over traditional checkpoint models.
In this blog post, we will explore what LoRA models are, how they work, where to find them, and how to use them in AUTOMATIC1111. Whether you're new to AI art or an experienced user, this guide will provide you with valuable insights into harnessing the power of LoRA models.
Article Keypoints
- Explore how Stable Diffusion Checkpoint merge works.
- The Origin of LoRA, and its advantages to Checkpoint merge by generating much smaller files, etc.
- Discuss the various ways to use LoRA locally.
Try out the Stable Diffusion Image Generator from Anakin AI 👇👇👇
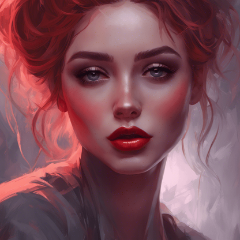
What are LoRA models?
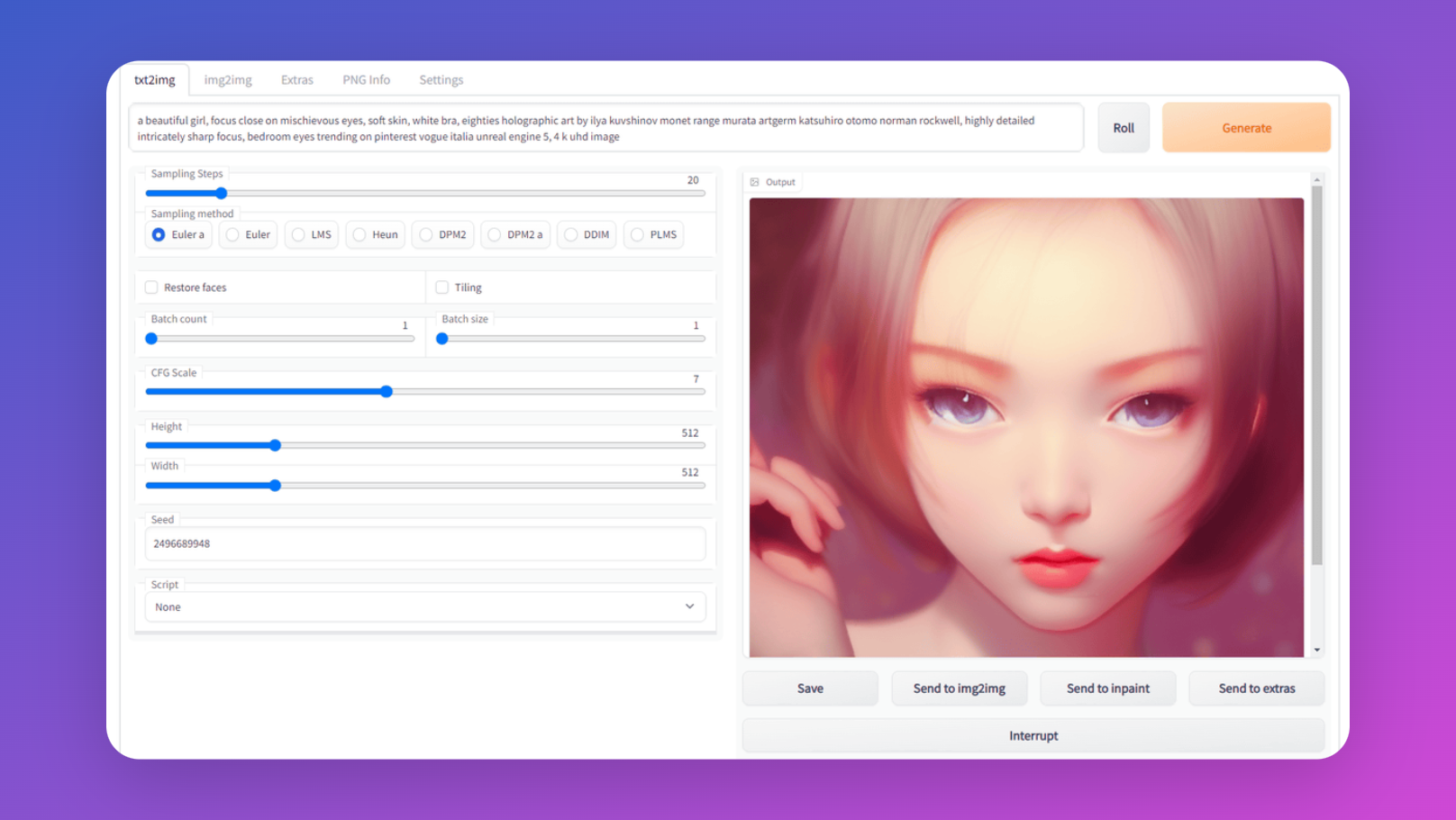
LoRA models, or Low-Rank Adaptation models, are a type of AI model that utilizes a low-rank adaptation technique to generate realistic and high-quality images. Compared to standard checkpoint models, LoRA models offer several advantages, including improved efficiency, enhanced flexibility, and the ability to produce more diverse and creative outputs.
How does LoRA work?
At a technical level, LoRA models incorporate low-rank adaptation into their neural networks. This technique allows the models to extract and utilize information from a wide range of images, resulting in more accurate and versatile artistic outputs. The use of low-rank adaptation also leads to faster convergence during the training process, making LoRA models more efficient and less resource-intensive.
Where to find LoRA models?
There are several sources where you can find LoRA models for use in AUTOMATIC1111. Two popular sources include Civitai and Hugging Face.
Civitai

Civitai is a well-known platform that offers a wide range of LoRA models for various AI applications. To find LoRA models on Civitai, simply navigate to their website and explore their collection of models. You can choose models based on your specific requirements and preferences.
Hugging Face
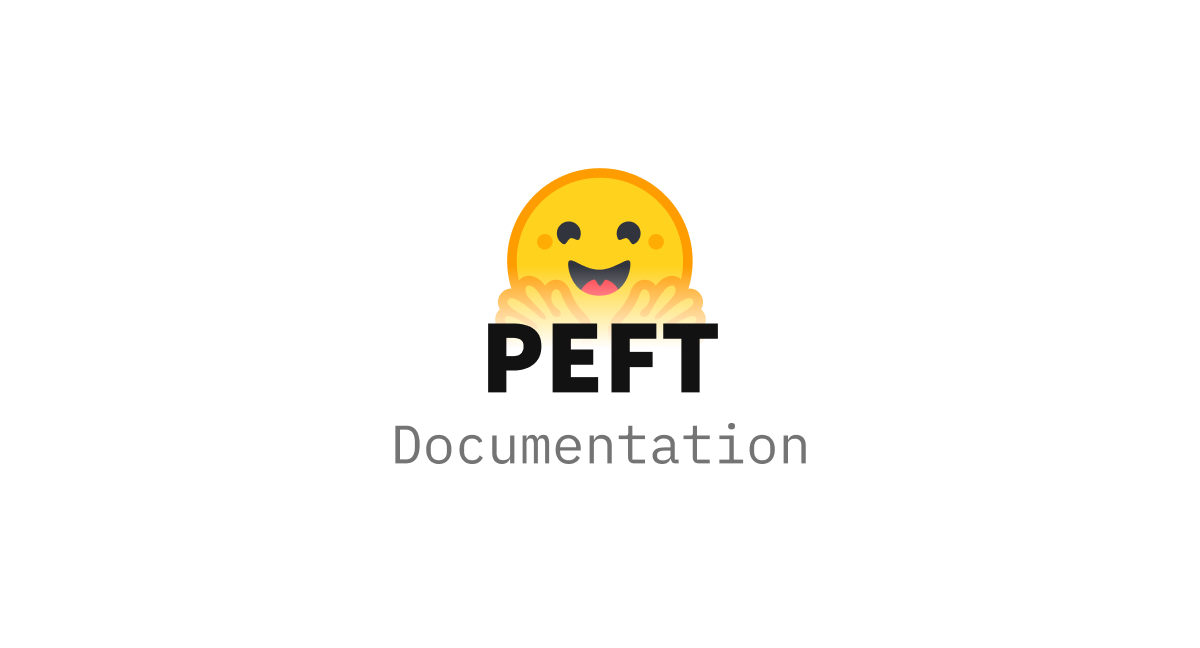
Hugging Face is another reputable repository for AI models, including LoRA models. By visiting Hugging Face's website, you can search for LoRA models and access the corresponding code and resources. Hugging Face is known for its user-friendly interface and extensive community support.
Checkpoint Merge in Stable Diffusion
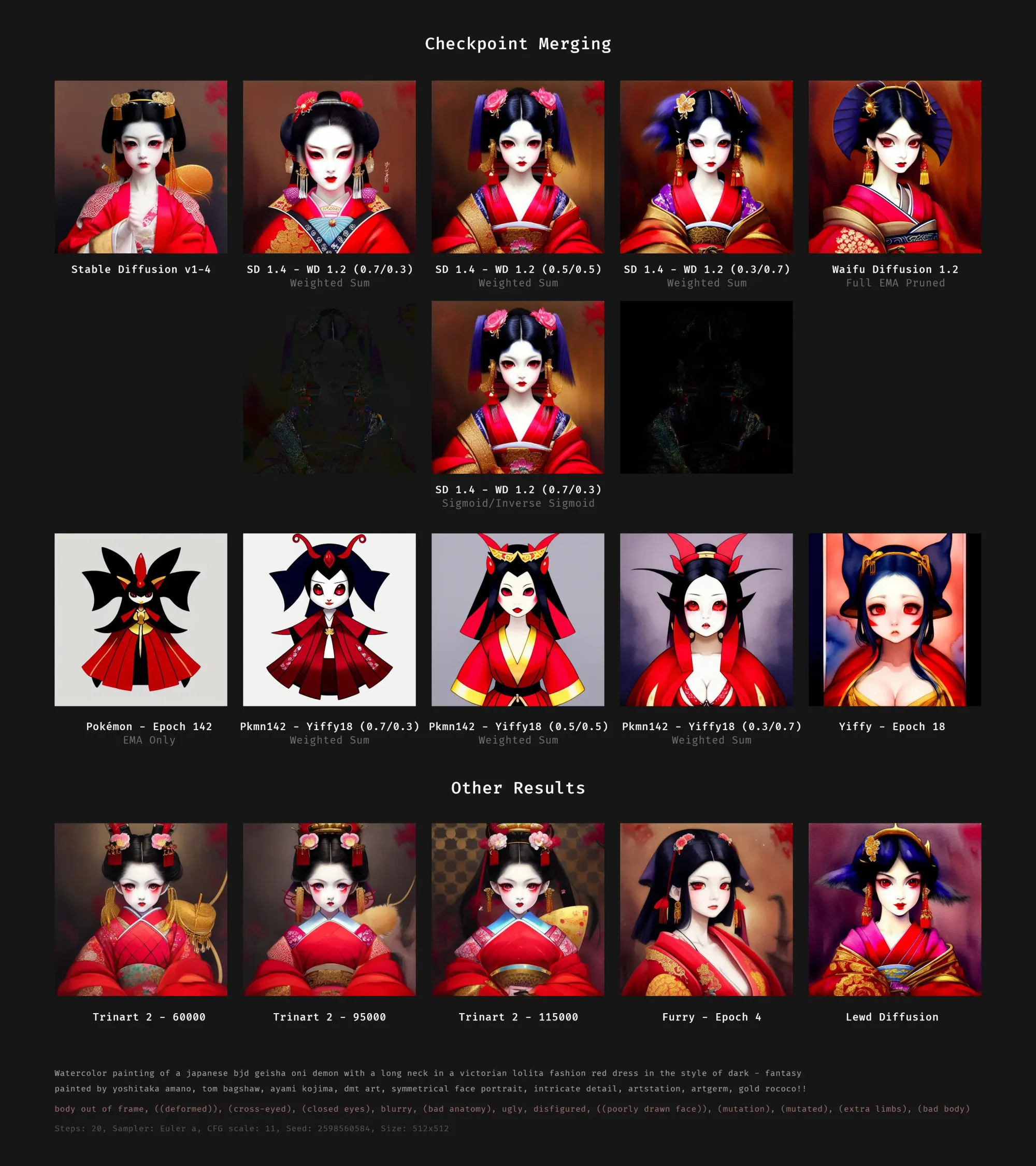
Checkpoint Merge in Stable Diffusion represents a sophisticated technique integral to advancing the capabilities of AI art generation models. This method is particularly significant in optimizing the performance and versatility of such models. Let’s delve deeper into this concept:
What is Checkpoint Merge:
- Conceptualization: In the realm of Stable Diffusion, Checkpoint Merge is not just about combining different training stages but integrating diverse learning experiences of the AI. Each checkpoint embodies a unique phase of the model's learning curve, capturing various nuances and styles of art generation.
- Strategic Enhancement: The primary goal of Checkpoint Merge is to amalgamate these distinct learning phases to create a model that exhibits a comprehensive understanding and ability in art generation. This synergy of checkpoints leads to a model that is not only proficient in a wide range of styles but also adapts more efficiently to new art generation tasks.
How to Perform Checkpoint Merge in Stable Diffusion:
Identifying Relevant Checkpoints:
- Criteria for Selection: The selection of checkpoints is critical and is based on factors like diversity in style representation, quality of output at each stage, and the uniqueness of learning exhibited.
- Balancing Act: Careful consideration is given to ensure that the selected checkpoints complement each other, covering a broad spectrum of artistic expressions.
Merging Process:
- Technical Approach: Utilizing advanced tools and scripts, the process involves aligning and integrating the neural networks of different checkpoints. This integration is done in a way that the strengths of one checkpoint compensate for the shortcomings of others.
- Iterative Refinement: The merged model often undergoes several iterations of refinement to ensure seamless integration of the various learning stages.
Testing and Validation:
- Quality Assurance: Rigorous testing is conducted to evaluate the artistic output, ensuring that the merged model adheres to high-quality standards and exhibits the desired artistic capabilities.
- Feedback Loop: Validation also includes a feedback mechanism, where the model’s output is continuously monitored and adjustments are made to refine its performance.
Pros and Cons of Checkpoint Merge in Stable Diffusion:
Pros:
- Comprehensive Capability: The merged model stands out in its ability to produce a vast array of artistic styles, effectively mimicking a collective artistic intelligence.
- Efficiency in Learning: Merging checkpoints often leads to a model that learns new styles and adapts to artistic challenges more swiftly.
Cons:
- Technical Expertise Required: The process demands a high level of technical know-how, particularly in understanding the nuances of neural network integration.
- Potential for Conflicting Traits: There’s a risk that certain traits or learning patterns of different checkpoints may conflict, leading to unpredictable or undesirable outputs.
Fine-Tuning/Training LoRA Models in Stable Diffusion: A Detailed Approach
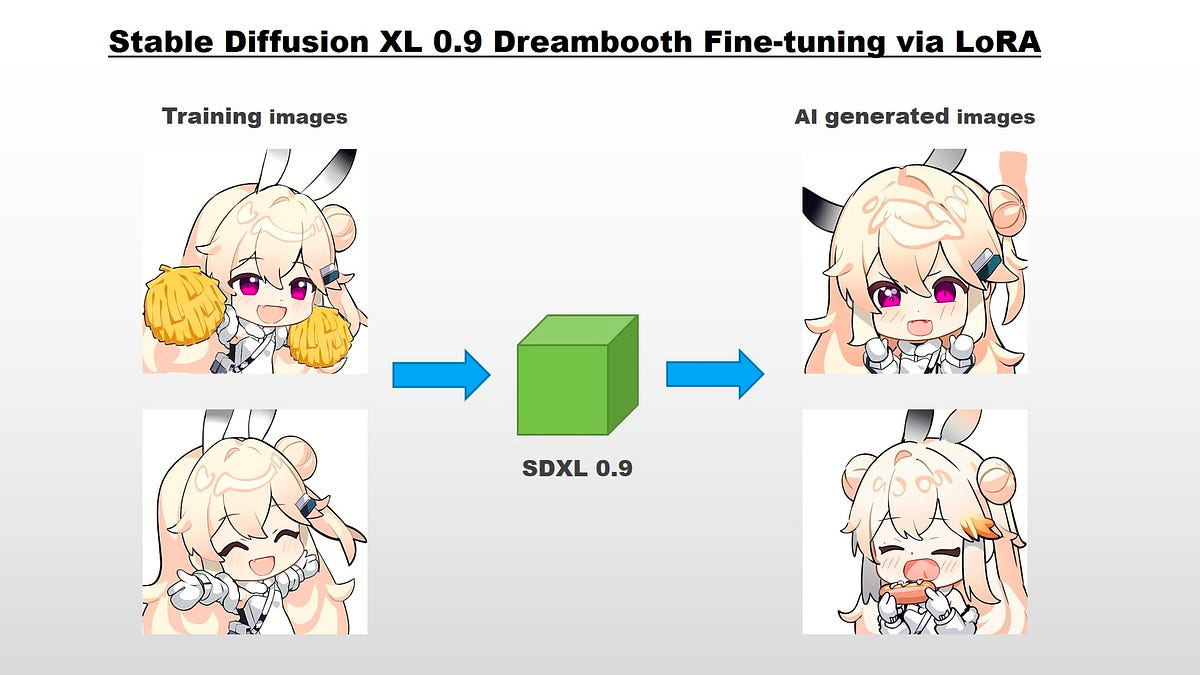
Fine-tuning, or training Low-Rank Adaptation (LoRA) models within Stable Diffusion offers a sophisticated method for customizing AI art generation. This process allows for the adaptation of the model to specific artistic styles or requirements. Here's an expanded view of each step in this process:
Understanding LoRA Fine-Tuning:
- Adaptive Learning: Fine-tuning a LoRA model is about making precise adjustments that enable the model to learn and reproduce specific artistic styles or characteristics with greater accuracy.
- Parameter Optimization: This involves tweaking the internal parameters of the model, which dictate how it interprets and processes artistic inputs. The goal is to enhance the model’s ability to generate images that closely align with the desired artistic vision.
Steps for Fine-Tuning LoRA:
Data Selection:
- Curating a Targeted Dataset: The first step involves carefully selecting a dataset that embodies the specific styles, themes, or characteristics desired in the output. This dataset serves as a training guide for the model.
- Diversity and Representation: Ensuring the dataset is diverse enough to provide a comprehensive learning experience for the model, covering various aspects of the chosen style.
Parameter Adjustment:
- Low-Rank Matrix Modification: At this stage, the low-rank matrices within the LoRA model are adjusted. These matrices are crucial as they determine how the model processes and generates artistic elements.
- Fine-Tuning for Specificity: Adjustments are made to align the model’s processing capabilities with the nuances of the selected dataset, ensuring the output reflects the desired artistic qualities.
Training:
- Re-Training Process: With the adjusted parameters, the model undergoes a re-training process using the curated dataset. This step is critical to embed the new artistic style into the model’s functioning.
- Monitoring and Adjustments: Continuous monitoring of the training process is essential to ensure that the model is adapting correctly. Adjustments are made as needed to refine the learning process.
Pros and Cons of Fine-Tuning LoRA Models:
Pros:
- Tailored Artistic Output: Fine-tuning allows for high customization, enabling the model to generate art that closely aligns with specific artistic visions or requirements.
- Efficient Learning Curve: Compared to training a model from scratch, LoRA models can be fine-tuned more quickly and efficiently, saving time and resources.
Cons:
- Computational Resources: The process is resource-intensive, requiring substantial computational power, especially when dealing with large datasets and complex artistic styles.
- Risk of Overfitting: There’s a risk that the model may become too narrowly focused on the specific dataset used for training, reducing its ability to generalize and create varied artistic outputs.
How to Install LoRA Models with Stable Diffusion
Try out the Stable Diffusion Image Generator from Anakin AI 👇👇👇
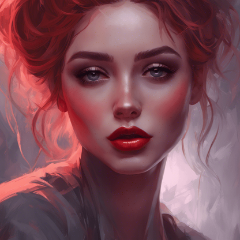
Step 1: Install the LoRA model
Before you can use a LoRA model in AUTOMATIC1111, you need to install it on your system.
Where to Put Lora in Stable Diffusion?
You can download your lora model from any source mentioned above, and save it in the following folder:
/stable-diffusion-webui/models/Lora
How to Use Lora with Stable Diffusion?
Once you have installed the LoRA model, it's time to integrate it into AUTOMATIC1111. AUTOMATIC1111 provides a user-friendly GUI that simplifies the process to generate stable diffusion arts. Here's how to set it up:
- Open AUTOMATIC1111 WebUI for Stable Diffusion: Launch AUTOMATIC1111 on your system.
- Specify the LoRA model: Click on the Lora Tab to select your downloaded Lora.
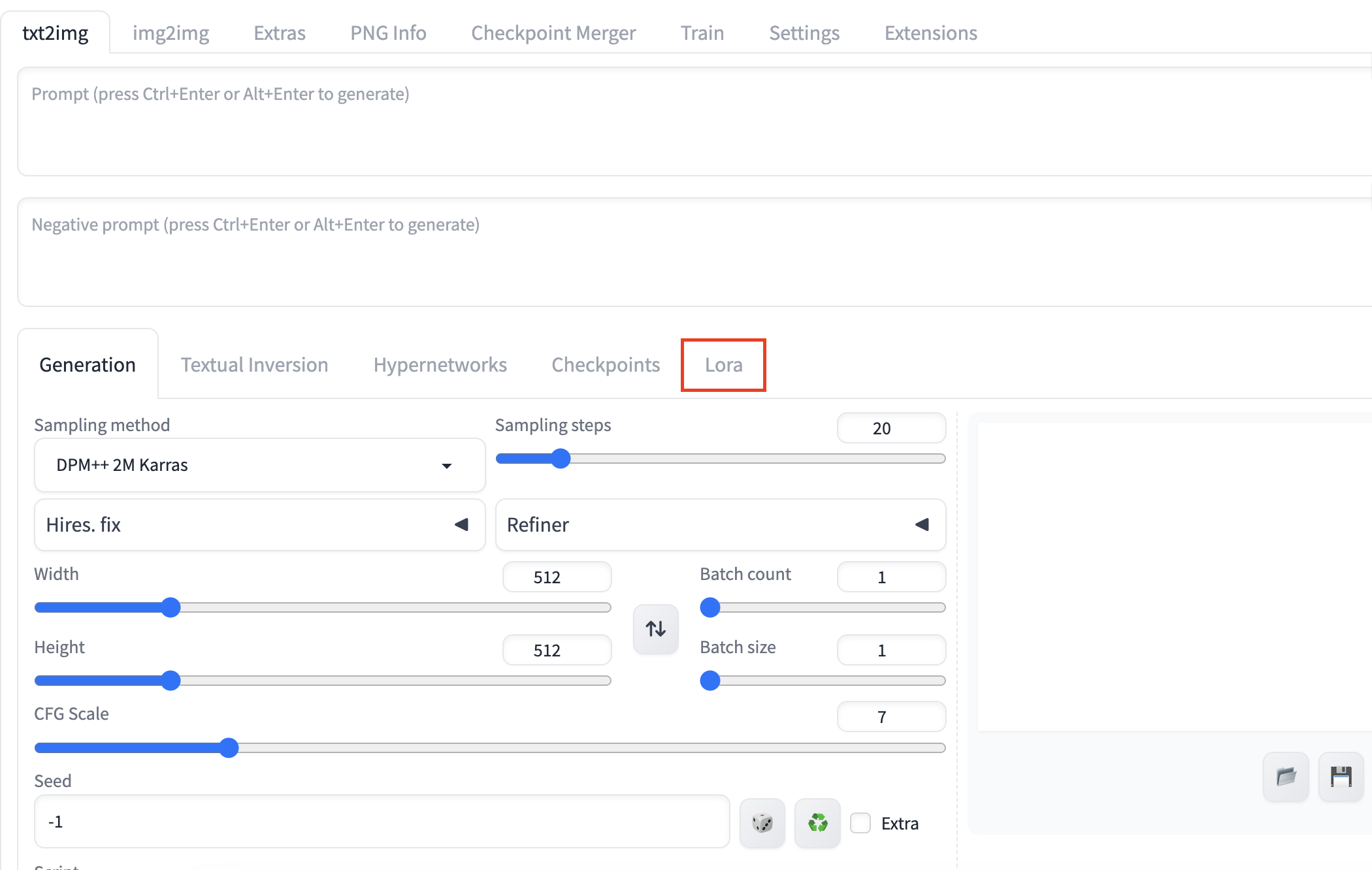
- Within the Lora tab, locate the option to select the model and choose the installed LoRA model from the available list.
- To use the Downloaded Lora, use the related "trigger words" for the given lora. Include this "Trigger Word" into your prompt to make it work.
For example:
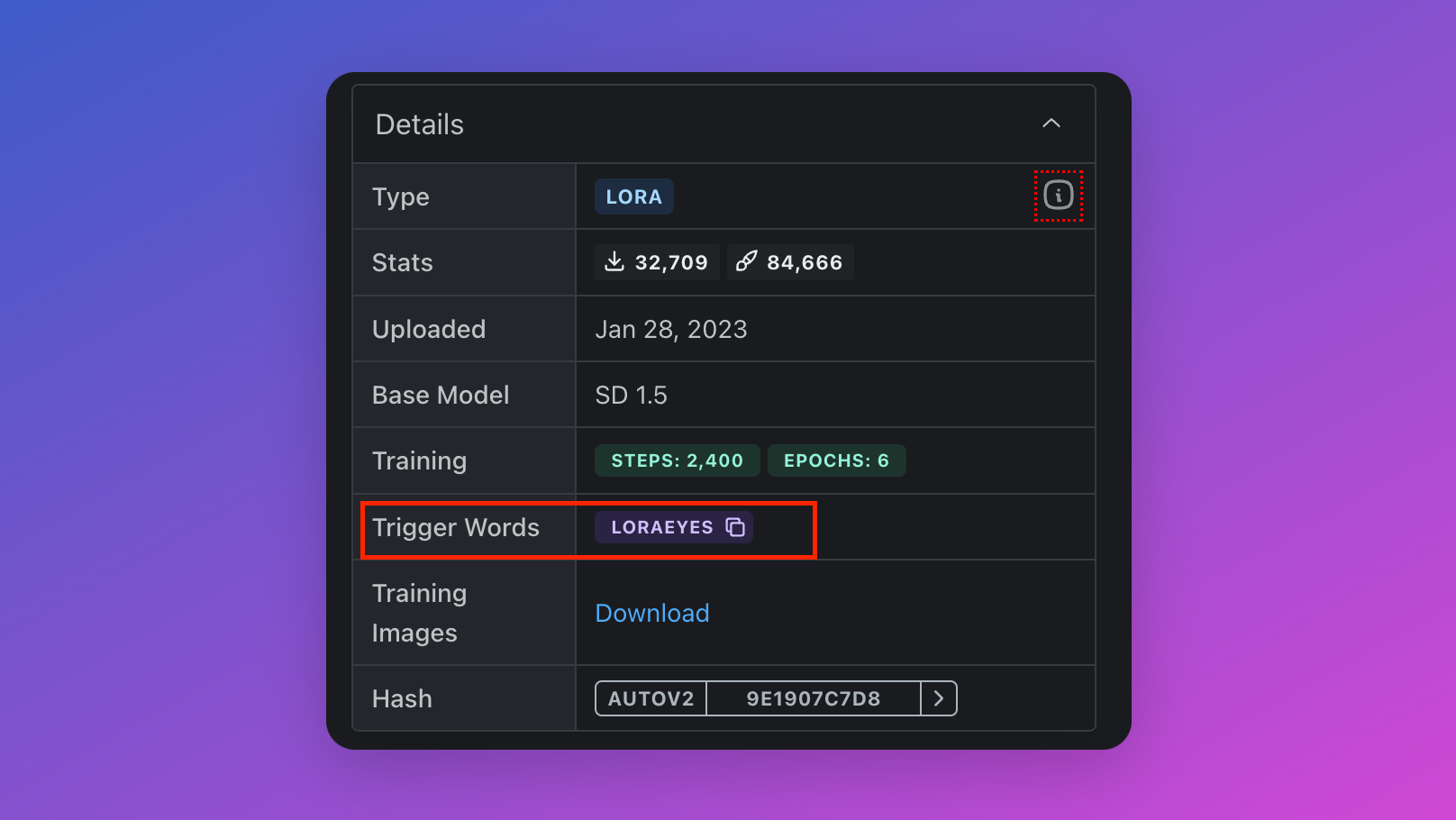
Can I use multiple LoRA models simultaneously?
Yes, you can use multiple LoRA models simultaneously in AUTOMATIC1111. This allows you to combine the artistic qualities of different models and achieve unique effects in your artwork.
How to update a LoRA model?
To update a LoRA model, you can visit the source from which you downloaded the model and check for any updates or new versions. Follow the instructions provided by the model source to update the model files in your system.
Future of LoRA Models in AI Art
As AI art continues to evolve, LoRA models are expected to play a significant role in shaping the landscape. Their ability to generate diverse and high-quality artworks opens up new possibilities for artists and creators. With ongoing research and advancements in AI, we can anticipate further enhancements to LoRA models, providing even more impressive outputs and expanding the boundaries of artistic expression.
Conclusion
LoRA models offer a powerful and flexible approach to AI art generation. By harnessing the low-rank adaptation technique, these models produce realistic and diverse outputs, enabling artists to explore new creative horizons. Through this comprehensive guide, we have explored what LoRA models are, how they work, where to find them, and how to utilize them in AUTOMATIC1111. Armed with this knowledge, we encourage you to experiment with LoRA models and unleash your artistic potential.
Try out the Stable Diffusion Image Generator from Anakin AI 👇👇👇
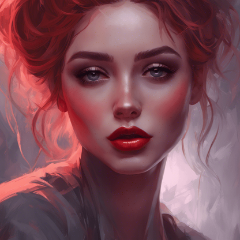