Step-Video-T2V represents a groundbreaking advancement in text-to-video generation, combining massive-scale neural architecture with innovative compression techniques to achieve state-of-the-art results. As an open-source model with 30 billion parameters, it pushes the boundaries of AI-generated video content through its unique technical implementations.
Want to create the best quality of AI Video with Minimax Hailuo AI, Runway ML, Luma AI, Hunyuan Video...?
Anakin AI rules them all! Use Anakin AI as the All-in-One Platform for all AI Video Models!
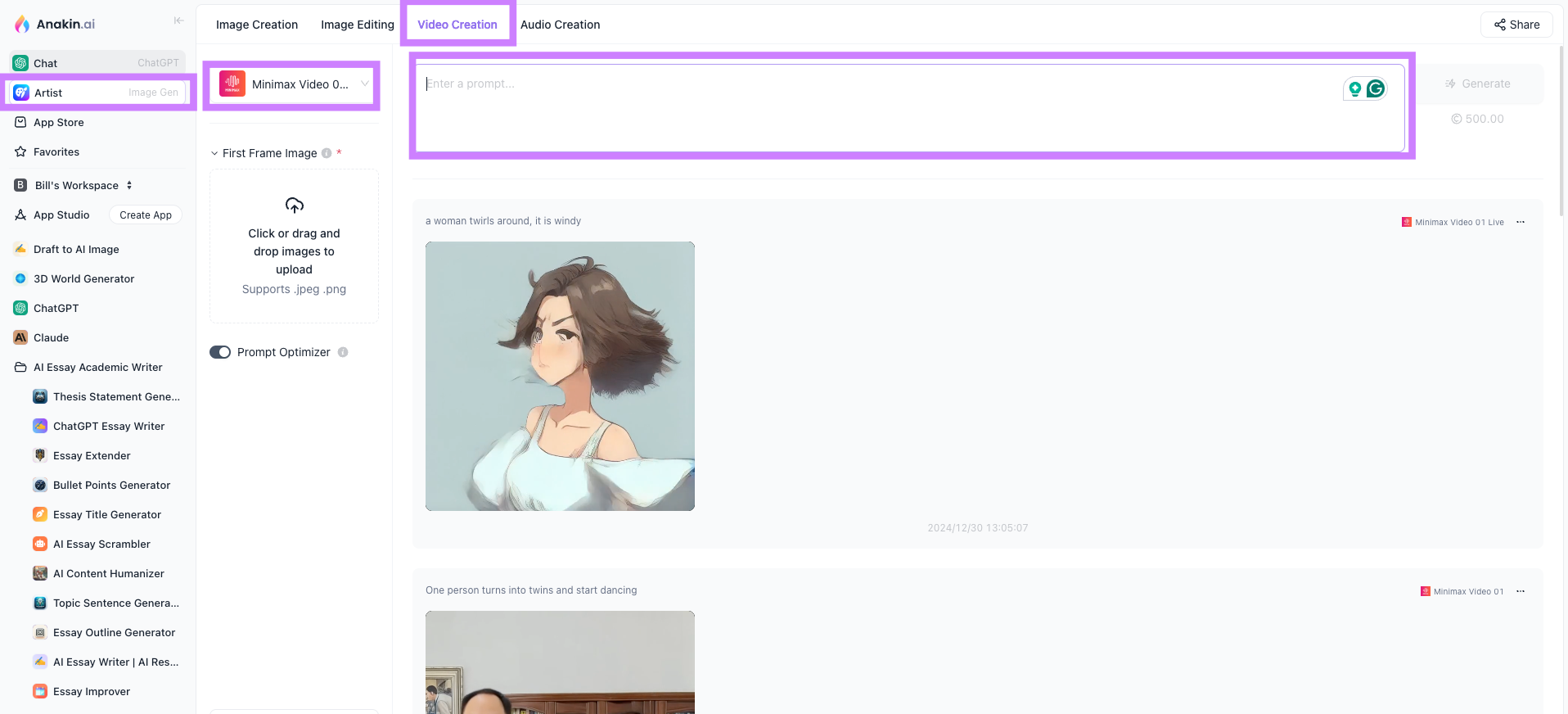
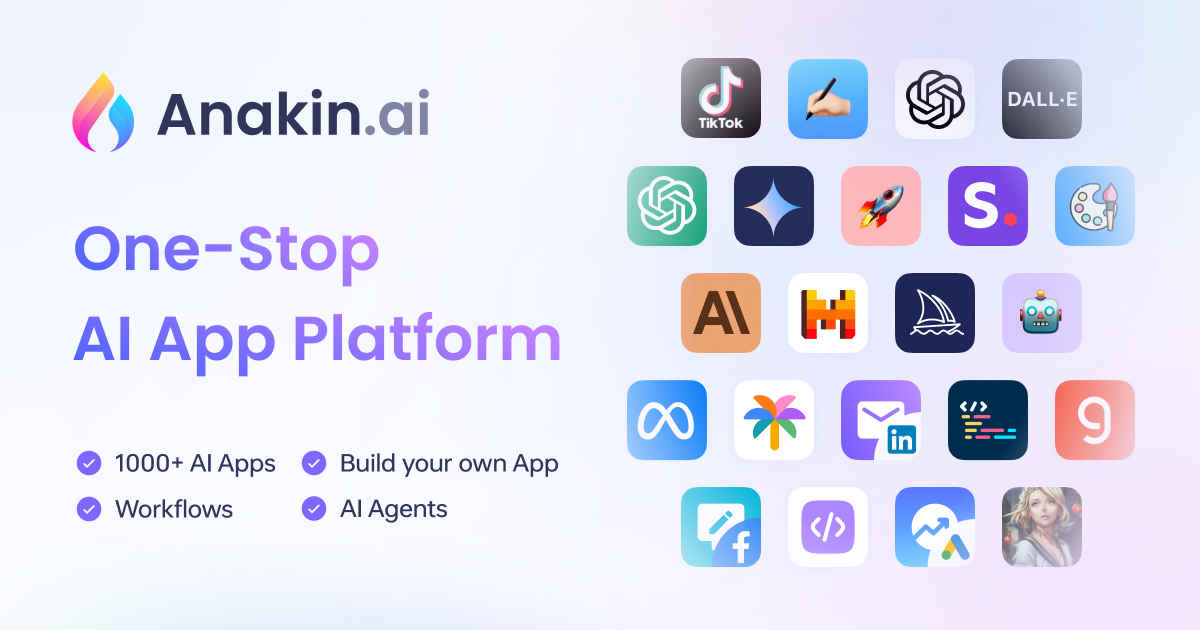
Step-Video-T2V Architectural Foundations
The model's architecture comprises three core components working in tandem:
Video-VAE Compression Engine
At the heart lies a deep compression Variational Autoencoder that achieves unprecedented 16x16 spatial and 8x temporal compression ratios. This enables:
- Latent space representation of 544x992 resolution videos
- Frame sequences compressed to 34x62 spatial dimensions
- Temporal compression reducing 204-frame videos to 25 latent steps
The VAE maintains reconstruction fidelity through novel quantization-aware training techniques while enabling efficient processing of long video sequences.
Diffusion Transformer (DiT) Backbone
A 48-layer transformer architecture employs:
- Full 3D attention mechanisms across spatial and temporal dimensions
- 48 attention heads with 128-dimensional embeddings per head
- 3D Rotary Position Embedding (RoPE) for sequence alignment
- QK-Norm stabilization for training stability
- Flow Matching objective function for noise prediction
Bilingual Text Encoding System
Dual text processors handle multilingual inputs:
- Hunyuan-CLIP - Bidirectional encoder for short prompts (<77 tokens)
- Step-LLM - Autoregressive encoder for complex/lengthy descriptions
The hybrid system supports nuanced understanding of both English and Chinese prompts through cross-lingual alignment.
Step-Video-T2V Training Methodology
The training pipeline employs a four-stage approach:
Text-to-Image Pre-training
- Initializes visual concept understanding
- Trains on 500M+ image-text pairs
- Establishes spatial relationship modeling
Text-to-Video Foundation Training
- Processes 10M video clips (3-15 seconds)
- Focuses on motion dynamics at 256x448 resolution
- Implements curriculum learning for stable convergence
Supervised Fine-Tuning (SFT)
- Uses 1M high-quality human-annotated videos
- Enhances aesthetic quality and prompt alignment
- Introduces style transfer capabilities
Direct Preference Optimization (DPO)
- Human feedback integration via pairwise comparisons
- Reduces visual artifacts by 37% (per benchmark metrics)
- Improves motion smoothness through reward modeling
The entire training process leverages a distributed infrastructure with:
- 4,096 NVIDIA H800 GPUs across multiple clusters
- Custom RPC framework (StepRPC) for cross-cluster communication
- Hybrid TCP/RDMA protocols achieving 98% bandwidth utilization
Step-Video-T2V Inference Characteristics
The model demonstrates unique operational requirements:
Hardware Specifications
- Minimum 4x NVIDIA A100/A800 GPUs (80GB VRAM)
- 743 seconds generation time for 204-frame videos (544x992)
- 77.64GB peak memory usage during inference
Optimization Techniques
- Decoupled text encoder/VAE/DiT processing
- Flash attention v2 acceleration
- Dynamic parallelism management
- Adaptive latent space caching
Key Inference Parameters
Parameter | Recommended Value |
---|---|
Inference Steps | 30-50 |
CFG Scale | 9.0 |
Time Shift | 13.0 |
Parallel Processes | 4-8 |
Step-Video-T2V Performance Metrics
Evaluation on the proprietary Step-Video-T2V-Eval benchmark reveals:
- 89% preference rate over commercial solutions in human evaluations
- 23% improvement in temporal consistency vs. previous SOTA
- 41 FVD score (Fréchet Video Distance)
- 0.82 CLIP-TScore for text-video alignment
The model particularly excels in:
- Complex camera motion synthesis
- Multi-object interaction scenarios
- Long-range temporal coherence (150+ frames)
- Cross-lingual prompt understanding
Step-Video-T2V Technical Challenges
Current limitations highlight research frontiers:
Physics Simulation
Struggles with accurate modeling of:
- Fluid dynamics (water flow, smoke)
- Rigid body collisions
- Light refraction/reflection
Compositional Understanding
Difficulties with rare concept combinations:
- "Penguin riding bicycle through desert"
- "Transparent car made of ice"
Computational Scaling
Training costs exceed $8M for full pipeline:
- 28 days on 4,096 GPUs
- 9.7 exaFLOP compute budget
Temporal Context
Maximum 204-frame (8.5s) generation limits:
- Narrative storytelling
- Gradual scene transitions
Step-Video-T2V Practical Applications
The open-source release enables diverse implementations:
Content Creation
- Automated video ads from product descriptions
- Social media clip generation
- Anime-style animation prototyping
Film Production
- Pre-visualization storyboards
- Background scene generation
- Special effects augmentation
Educational Tools
- Historical event reenactments
- Scientific process visualization
- Language learning through situational videos
Research Platforms
- Baselines for video understanding models
- Testbed for new compression algorithms
- Benchmark for distributed training systems
Conclusion
Step-Video-T2V establishes new technical standards for open-source video generation through its innovative integration of massive-scale transformers, advanced compression techniques, and human-aligned optimization strategies. While current limitations in physics modeling and computational demands persist, the model's architectural innovations and open availability provide a crucial foundation for future advancements in dynamic visual synthesis. As the community builds upon this work, we anticipate rapid progress toward more efficient, accessible, and capable video generation systems.