Open Source LLM is getting so hot now. Wherever you go, you start to hear these confusing names of the latest Open Source LLMs people are quietly whispering. However, as fun as they are, they are not yet reaching the crown, GPT-4.
Until now. Starling-7B, a new player steps into the spotlight. As a beacon of open-source innovation, it's quickly becoming a notable rival to the well-known GPT-4. This emerging technology is not only a technological marvel but also a symbol of communal effort in the AI field.
Anakin AI can help you easily create any AI app with highly customized workflow, with access to Many, Many AI models such as GPT-4-Turbo, Claude-2-100k, API for Midjourney & Stable Diffusion, and much more!
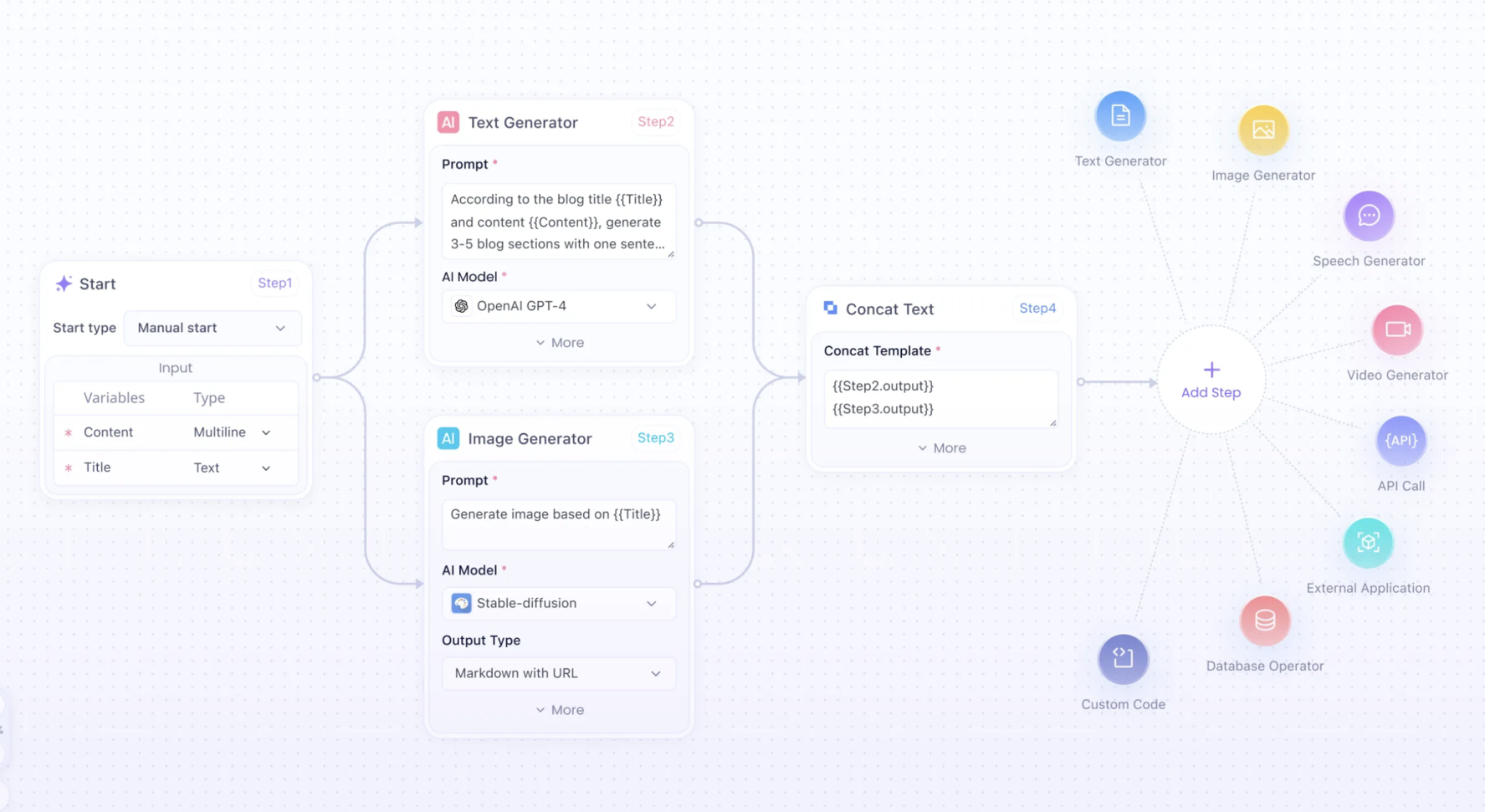
Interested? Check out Anakin AI and test it out for free!๐๐๐
What is Starling-7B?
Starling-7B is an open-source Large Language Model (LLM) making headlines for its remarkable performance and the collaborative ethos underpinning its development. The model employs Reinforcement Learning from AI Feedback (RLAIF) and utilizes a specially curated dataset named Nectar. Significantly, Starling-7B boasts an MT Bench score of 8.09, placing it just behind GPT-4 in terms of capabilities, which is particularly notable considering its open-source nature.
Starling-7B is a testament to the potential of community-driven AI research. It stands out for several reasons:
- Open-source model: Unlike proprietary models, Starling-7B opens up possibilities for wider experimentation and improvement by the research community.
- Performance: With scores close to GPT-4, it shows that open-source models can compete with industry leaders.
- Innovation: The model's training involves new techniques like RLAIF, pushing the boundaries of AI research.
Starling-7B Benchmark: Is It Really Better Than GPT-4?
Evaluating the efficacy of LLMs like Starling-7B involves intricate benchmarks that assess various aspects of the model's abilities. Here's the radar chart:
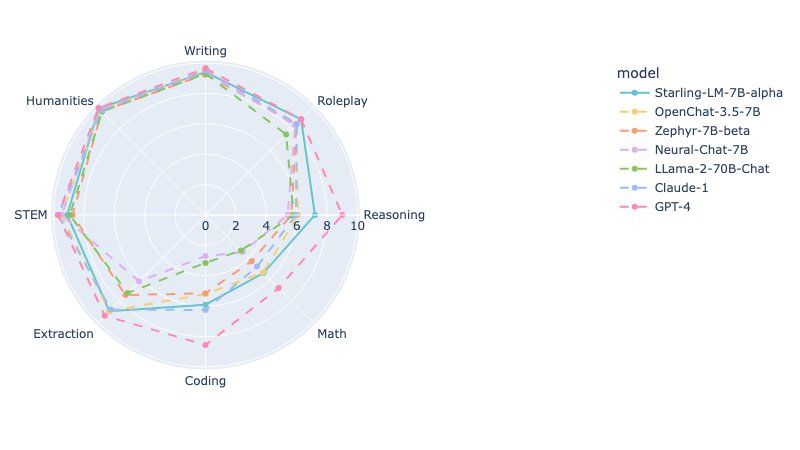
The radar chart reveals how Starling-7B stacks up against other notable models across various competencies:
- Writing and Humanities: Starling-7B exhibits robust performance, similar to models like GPT-4 and Claude-1, indicating its strong capability in understanding and generating humanistic content.
- Reasoning and Roleplay: It is on par with models like Llama-2-70B-Chat in reasoning, but there is room to improve in roleplay, where GPT-4 leads.
- STEM and Coding: Starling-7B shows promise, but models like GPT-4 and Neural-Chat-7B demonstrate superior performance in these technical areas.
- Math: This is an area where Starling-7B has room to grow, as GPT-4 and Zephyr-7B-beta outperform it.
- Extraction: Its ability to extract information from texts is competitive, though not quite at the level of OpenChat-3.5-7B and GPT-4.
These insights help guide further development and training for Starling-7B to close the gap in areas like Math and STEM and maintain its strong points in Writing and Humanities.
The table below illustrates Starling-7B's performance compared to its contemporaries:
Model | MT Bench | AlpacaEval | MMLU | Key Features |
---|---|---|---|---|
GPT-4-Turbo | 9.32 | 97.70 | ? | Top performer with unknown tuning method |
GPT-4 | 8.99 | 95.28 | 86.4 | High performance with SFT + PPO tuning |
Starling-7B | 8.09 | 91.99 | 63.9 | Close to GPT-4, community-driven |
Claude-2 | 8.06 | 91.36 | 78.5 | Comparable performance with unknown tuning |
GPT-3.5-Turbo | 7.94 | 89.37 | 70 | Previous version with room for improvement |
Note: MMLU (Massive Multitask Language Understanding) scores are not available for all models.
Starling-7B's performance in these benchmarks is not the endpoint but a step in its evolutionary journey. As its training continues and its dataset grows, we can anticipate improvements in its benchmark scores and, consequently, its capabilities and applicability in real-world scenarios.
Below is the Hugging Face Card for Starling-LM-7B-alpha model.

How Is Starling-7B Trained?
At the heart of Starling-7B's functionality is RLAIF, a training method that combines the strengths of supervised learning and reinforcement learning. This hybrid approach is designed to refine the model's responses to be more helpful and less prone to producing harmful content.
The process involves several steps:
- Gathering Data: Starling-7B relies on Nectar, a dataset with over 183K chat prompts and 3.8 million pairwise comparisons, to train its reward model.
- Refining Responses: Using RLAIF, Starling-7B evaluates responses for helpfulness and safety, learning to prefer responses that score higher on these metrics.
- Benchmarking: The model's performance is continually tested against benchmarks like MT Bench and AlpacaEval to ensure improvements align with human preferences.
Training with Nectar Dataset
The Nectar dataset is the backbone of Starling-7B's training regime. It's a collection of chat prompts and responses, carefully labeled to provide the model with a nuanced understanding of human conversation. This rich dataset allows Starling-7B to:
- Analyze various conversation styles and topics.
- Learn from a diverse range of responses to improve its own.
- Develop a sense of context and appropriateness for its replies.
Reinforcement Learning from AI Feedback (RLAIF)
RLAIF is what propels Starling-7B beyond a mere mimic of human language to a model that can genuinely assist and engage with users. This process involves:
- Assessing the quality of responses based on predefined criteria.
- Adjusting the model's parameters to favor responses that meet these standards.
- Iteratively improving through cycles of feedback and tuning.
This rigorous training process equips Starling-7B with the ability to handle real-world tasks more effectively and safely.
No, Starling-7B is not immune to prompt injection
While Starling-7B marks a significant stride in the open-source AI realm, it's not without its challenges, particularly when it comes to prompt injection, a form of AI manipulation. Here's a concise look at its strengths and challenges:
Strengths:
- Performance: Close to GPT-4's with an MT Bench score of 8.09.
- Open-source: Encourages community contributions and transparency.
- Innovation: Utilizes RLAIF for continuous improvement.
Challenges:
- Complex Reasoning: Difficulty with tasks requiring advanced problem-solving.
- Real-World Application: Adapting to the unpredictability of real-life usage.
- Jailbreaking: Vulnerable to prompts aimed at undermining its rules.
Aspect | Strengths | Challenges |
---|---|---|
Learning | Gains from diverse interactions and feedback. | Struggles with complex reasoning and math. |
Adaptability | Improved through community-driven development. | Faces a gap in handling real-world scenarios. |
Safety | Trained for harmlessness and helpfulness. | Susceptible to jailbreaking and prompt injection. |
Despite its capabilities, it's clear that prompt injection โ crafting inputs to elicit unintended behaviors โ remains an issue for Starling-7B. The AI community is working on:
- Robust Safety Measures: Enhancing Starling-7B's ability to recognize and resist harmful prompts.
- Data Diversification: Ingesting varied datasets to improve response accuracy and appropriateness.
- Community Vigilance: Leveraging open-source collaboration to identify and patch vulnerabilities swiftly.
Starling-7B's journey is a testament to the dynamic nature of AI development โ a balance of pushing the envelope while safeguarding against exploitation. Its open-source status plays a pivotal role in this, as it invites a broad spectrum of developers to aid in fortifying the model against prompt injection and other forms of AI manipulation.
Conclusion: The Future of LLM with Starling-7B
The future of Starling-7B is as open as its source code. With a strong foundation and a community-driven approach, the potential for growth and innovation is immense. As it stands, Starling-7B represents not just a technological alternative to giants like GPT-4 but also a vision of collaborative, transparent, and ethical AI development.
As we look ahead, the model's trajectory will likely include more diverse data, sophisticated training methodologies, and broader community engagement, setting the stage for an exciting era in the world of AI.
FAQs
How does Starling-7B differ from GPT-4?Starling-7B is an open-source model that employs RLAIF and operates closely to GPT-4's performance, particularly in benchmarks like MT Bench.
Can anyone use the Starling-7B model?Yes, as an open-source model, Starling-7B is accessible for research and non-commercial use.
What is the Nectar dataset?Nectar is a GPT-4 labeled ranking dataset used to train Starling-7B, featuring a wide array of chat prompts and responses.
Is Starling-7B better at understanding human language than other models?Starling-7B is designed to be highly competent in understanding and engaging in human language, as evidenced by its performance scores.
How does Starling-7B contribute to AI safety?Starling-7B is trained with a focus on helpfulness and harmlessness, utilizing benchmarks that assess safety and interaction quality.