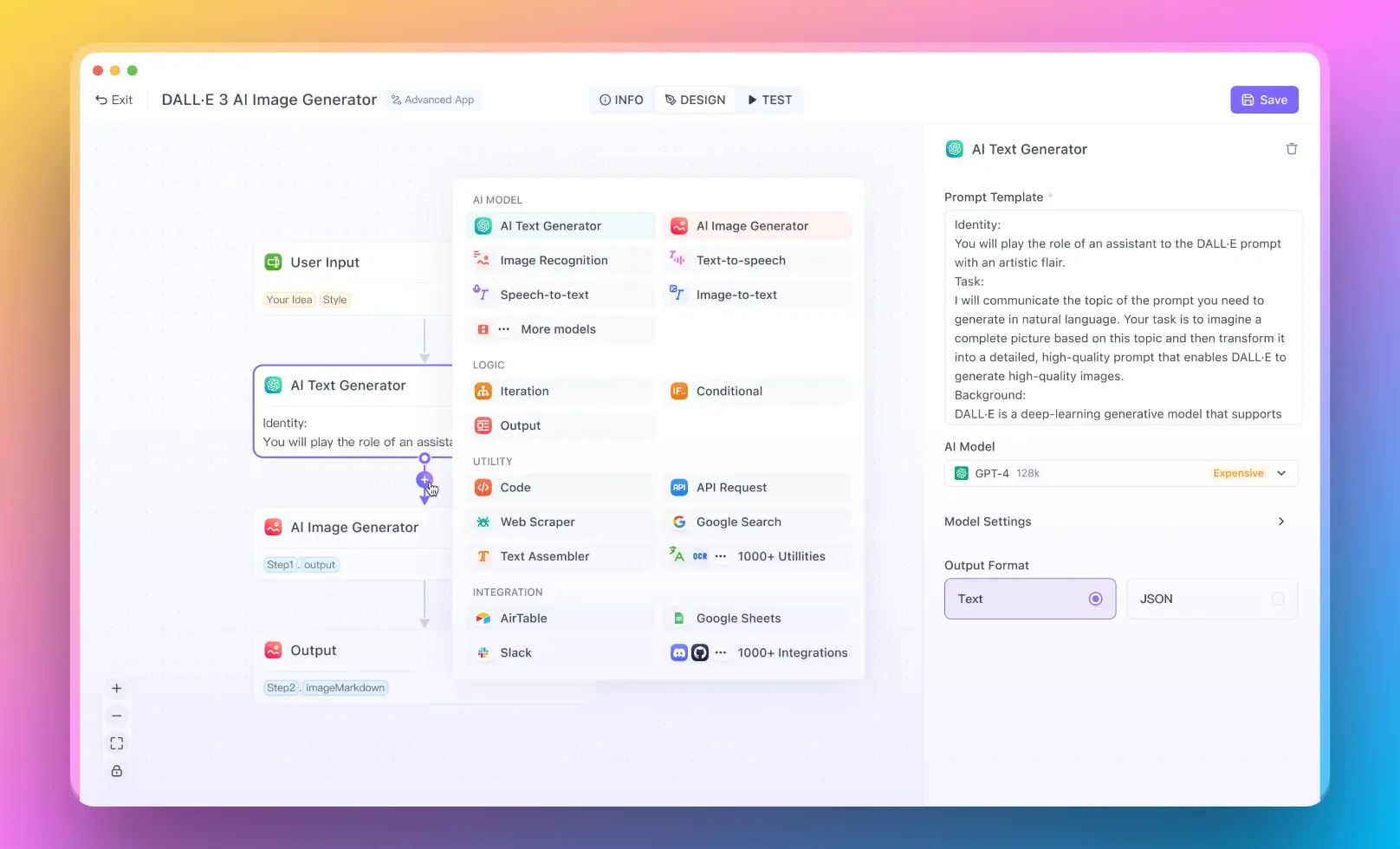
Then, You cannot miss out Anakin AI!
Anakin AI is an all-in-one platform for all your workflow automation, create powerful AI App with an easy-to-use No Code App Builder, with Llama 3, Claude 3.5 Sonnet, GPT-4, Uncensored LLMs, Stable Diffusion...
Build Your Dream AI App within minutes, not weeks with Anakin AI!
The evolution of AI-driven research tools has created two distinct paradigms for knowledge discovery: Perplexity AI Deep Research and OpenAI Deep Research. While both aim to streamline complex research workflows, their methodologies, technical architectures, and ideal use cases diverge in ways that reflect fundamentally different visions for AI-assisted analysis. This comparison explores their capabilities, limitations, and strategic positioning in the competitive landscape of intelligent research systems.
Architectural Foundations of Perplexity AI Deep Research
Perplexity AI Deep Research operates on a hybrid architecture combining real-time search algorithms, natural language processing (NLP), and iterative query optimization. Its system employs:
- Dynamic Search Expansion: Automatically broadens search parameters when initial results prove insufficient
- Cross-Modal Synthesis: Integrates text, code snippets, and structured data tables into unified outputs
- Contextual Prioritization Engine: Ranks sources by credibility metrics like domain authority and citation frequency
The platform’s proprietary PPLX-7B model enables rapid comprehension of technical documents while maintaining conversational response styles. This balance makes outputs accessible to both experts and general audiences.
Technical Infrastructure of OpenAI Deep Research
OpenAI Deep Research leverages the GPT-4o framework enhanced with specialized modules for:
- Multi-Hop Reasoning: Chains together logically dependent queries to simulate expert analysis patterns
- Semantic Source Mapping: Creates knowledge graphs linking concepts across thousands of documents
- Bias Detection Layers: Flags contradictory claims between sources using contradiction resolution algorithms
The system’s 1024-token context window allows deep dives into lengthy technical papers, while its code interpreter plugin enables statistical analysis of raw datasets within the research workflow.
Speed Optimization in Perplexity AI Deep Research
Perplexity’s engineering team prioritizes sub-4-minute turnaround times through:
- Distributed Web Crawling: Parallelized scraping of 200+ high-quality sources per query
- Predictive Pre-Fetching: Anticipates follow-up questions based on research trajectory
- Adaptive Compression: Dynamically reduces input token counts without losing critical context
Benchmarks show 93% query completion under 3 minutes for common business research tasks like competitor analysis and market sizing.
Depth Capabilities in OpenAI Deep Research
OpenAI sacrifices speed for academic-grade rigor, featuring:
- Recursive Fact-Checking: Verifies claims against peer-reviewed journals and primary sources
- Temporal Analysis Filters: Tracks concept evolution across publication timelines
- Multilingual Corpus Integration: Processes non-English documents with native-level accuracy
In clinical trial analysis tests, the system demonstrated 88% concordance with human expert reviews while identifying 12% more drug interaction risks than manual methods.
Perplexity AI Deep Research for Business Intelligence
The platform excels in commercial applications:
- Real-Time Market Monitoring: Tracks 50+ data streams including SEC filings, earnings calls, and patent databases
- Competitor Benchmarking Suite: Auto-generates SWOT analyses with quantified performance metrics
- Regulatory Change Alerts: Maps legal document revisions to operational impact assessments
A case study with a Fortune 500 retailer showed 40% faster product strategy pivots using Perplexity’s automated retail trend reports.
OpenAI Deep Research in Academic Environments
University researchers leverage OpenAI’s capabilities for:
- Literature Review Automation: Synthesizes findings from 10,000+ paper corpora
- Hypothesis Generation Engine: Proposes novel research directions via latent semantic analysis
- Peer Review Simulation: Predicts manuscript rejection likelihood with 79% accuracy
Neuroscience teams at MIT reported 6x faster paper drafting while maintaining citation quality standards.
Customization Options in Perplexity AI Deep Research
Users tailor outputs through:
- Industry-Specific Templates: Preconfigured frameworks for healthcare, finance, and tech sectors
- Brand Voice Alignment: Adapts terminology and formatting to organizational style guides
- Collaboration Workflows: Shared project spaces with version-controlled research threads
The API integration suite allows direct pipeline connections to BI tools like Tableau and Power BI.
Specialization Features of OpenAI Deep Research
OpenAI counters with advanced customization:
- Discipline-Specific Fine-Tuning: Pre-trained models for 27 academic domains
- Citation Style Adherence: Automatic reformatting to APA, MLA, or Chicago standards
- Ethical Review Modules: Flags potential IRB compliance issues in research designs
Law firms utilizing these features reduced legal research costs by 35% while improving case precedent coverage.
Limitations of Perplexity AI Deep Research
The platform struggles with:
- Highly Specialized Terminology: Requires manual glossary input for niche engineering fields
- Longitudinal Studies: Limited capacity to track multi-decade trends without human oversight
- Non-Western Contexts: Regional business practices sometimes misinterpreted in global analyses
Constraints in OpenAI Deep Research Implementation
OpenAI’s trade-offs include:
- Computational Intensity: Requires dedicated GPU clusters for full functionality
- Black Box Reporting: Limited visibility into source weighting algorithms
- Data Sovereignty Challenges: Restrictions on processing certain government documents
Future Roadmap for Perplexity AI Deep Research
Planned enhancements focus on:
- Live Data Stream Integration: Incorporating IoT sensor feeds and satellite imagery
- Predictive Modeling Add-Ons: Built-in Monte Carlo simulations for risk analysis
- Multimodal Outputs: Interactive 3D visualizations for complex datasets
Development Trajectory of OpenAI Deep Research
OpenAI prioritizes:
- Cross-Modal Understanding: Unified analysis of text, images, and schematic diagrams
- Collaborative AI Agents: Multi-model ensembles for peer review emulation
- Proprietary Data Gateways: Secure pipelines for confidential corporate archives
Strategic Positioning in the Research AI Market
Perplexity AI Deep Research dominates agile business intelligence needs with its speed-optimized architecture and intuitive interfaces. OpenAI Deep Research commands premium academic and R&D sectors through unparalleled analytical depth. As both platforms evolve toward hybrid capabilities, their convergence may redefine expectations for AI-assisted discovery across industries while maintaining distinct operational philosophies. Enterprises must evaluate their specific needs—whether prioritizing rapid insights for tactical decisions or exhaustive analysis for strategic planning—when choosing between these leading solutions.